A Future of Personalized Entertainment AI Recommender Systems

The entertainment industry has experienced a significant shift with the advent of artificial intelligence (AI) recommender systems. These intelligent algorithms have revolutionized the way we discover and consume content, providing a personalized experience that caters to our unique preferences. In this article, we will delve into the various aspects of AI recommender systems and explore their implications for the future of entertainment.
1. Enhanced Discovery
AI recommender systems excel at understanding our individual tastes and interests, allowing us to discover new content that we may have otherwise overlooked. By analyzing our previous viewing or listening habits and comparing them to a vast database of content, these systems can recommend highly relevant options. This not only saves time but also introduces users to a diverse range of entertainment choices.
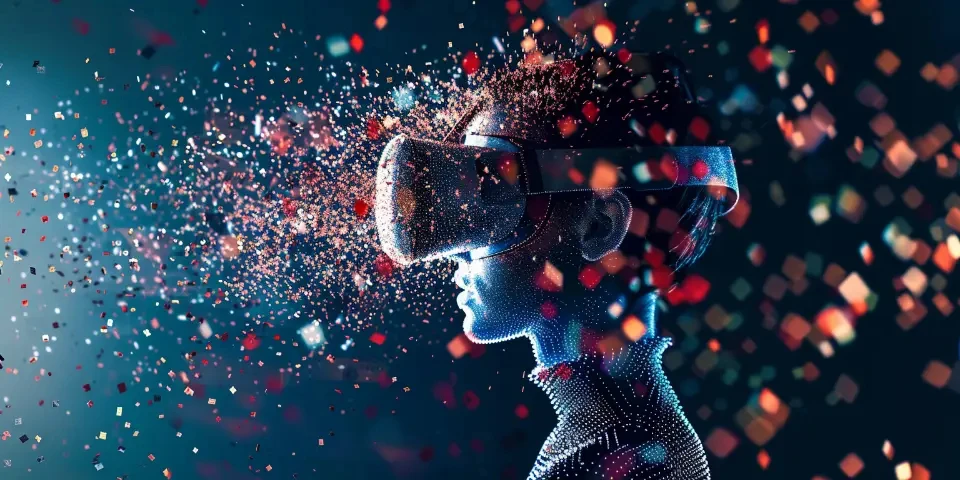
For instance, Netflix's recommender system uses a combination of machine learning and collaborative filtering techniques to suggest movies and TV shows based on a user's viewing history and preferences. This personalized approach has significantly improved the accuracy of content recommendations, making it easier for users to explore new genres or discover hidden gems.
2. Customized User Interfaces
AI recommender systems have the potential to reshape the user interface of entertainment platforms. These systems can analyze an individual's preferences, browsing patterns, and even physiological responses to optimize the layout and design of the interface. This customization enhances user experience by presenting the most relevant content upfront and reducing clutter.
For example, Spotify's discover weekly playlist utilizes AI algorithms to curate a personalized collection of songs based on a user's listening history and preferences. The user interface of the app is then tailored to prioritize these recommendations, making it effortless for users to find new music.
3. Dynamic Content Curation
AI recommender systems not only help users discover content but also enable dynamic and personalized curation of playlists, movie queues, or news feeds. These systems can adapt to user preferences and behaviors, continuously updating and refining recommendations as users engage with the platform.
Pandora, a popular music streaming service, employs an AI-powered recommender system that learns from user feedback to create customized radio stations. As users provide feedback on songs they like or dislike, the system refines its understanding of their musical preferences and tailors future recommendations accordingly.
4. Cross-Platform Recommendations
AI recommender systems are not limited to a single entertainment platform. They have the potential to provide cross-platform recommendations, enabling users to seamlessly discover content across multiple services.
Google Discover is an AI-powered recommender system that curates personalized news articles, videos, and other content from various sources based on a user's interests and search history. By providing recommendations from a wide range of publishers, this system ensures that users can access diverse content without needing to navigate through multiple platforms.
5. Improved Customer Retention
Personalized recommendations play a crucial role in customer retention for entertainment platforms. By providing users with content that aligns with their interests, AI recommender systems increase engagement, leading to higher satisfaction and a reduced likelihood of churn.
Amazon Prime Video's recommender system uses machine learning algorithms to analyze user behavior and preferences. Based on this analysis, tailored recommendations are provided, which have been found to significantly increase user engagement and retention on the platform.
6. Ethical Considerations
While AI recommender systems offer tremendous benefits, ethical concerns also come into play. There is a risk of creating filter bubbles, where users are only exposed to content that reinforces their existing beliefs or interests. This can limit exposure to diverse perspectives and hinder personal growth.
Platforms need to develop algorithms that strike a balance between personalization and avoiding excessive content filtering. OpenAI's GPT-3, an AI language model, aims to address this by allowing users to control the level of filtering in recommendations, ensuring a more diverse and well-rounded experience.
7. Privacy and Data Security
AI recommender systems heavily rely on user data to provide accurate recommendations. However, this raises concerns about privacy and data security. It is essential for entertainment platforms to implement robust security measures to protect user information and ensure transparency regarding data usage.
Furthermore, users should have the option to control the extent to which their data is used for recommendation purposes. Platforms like YouTube and Spotify have taken steps in this direction by allowing users to modify their recommendation settings or view and delete their data history.
FAQs
Q1: Can AI recommender systems adapt to changing preferences?
A1: Yes, AI recommender systems continually learn and refine recommendations based on user feedback and interactions. As preferences change over time, the system adapts to provide up-to-date and relevant recommendations.
Q2: How do AI recommender systems handle diverse tastes within a household?
A2: Some AI recommender systems allow multiple user profiles within a single account. Each profile can have its preferences, ensuring that recommendations are personalized for each individual within the household.
Q3: Are AI recommender systems limited to entertainment content only?
A3: No, AI recommender systems can be applied to various domains beyond entertainment, such as e-commerce, news, or social media. The underlying algorithms can be tailored to specific contexts to provide personalized recommendations in different areas.
References:
1. Chen, H., & Pu, P. (2019). Personalized Recommender Systems in the Entertainment Domain. Springer.
2. Aggarwal, C. C., & Zhang, N. (2020). Recommender Systems: The Textbook. Springer.
3. Spotify. (n.d.). Discover Weekly. Retrieved from: https://www.spotify.com/us/discoverweekly/
Explore your companion in WeMate