Accelerating Financial Fraud Detection with Spark AI

Financial fraud has become a major concern for businesses and individuals alike. As technology evolves, so do the methods used by fraudsters, making it increasingly challenging to detect and prevent fraudulent activities. However, with the emergence of Spark AI, financial institutions now have a powerful tool at their disposal to accelerate the detection and mitigation of financial fraud. In this article, we will explore how Spark AI revolutionizes fraud detection and why it is essential in the fight against financial crimes.
1. Real-time Transaction Monitoring
Traditional fraud detection systems often analyze transactions offline, leading to delays in identifying and stopping fraudulent activities. Spark AI enables real-time transaction monitoring, allowing financial institutions to detect and respond to suspicious transactions as they occur. By leveraging Spark's distributed computing capabilities and machine learning algorithms, fraud patterns can be identified swiftly, minimizing the potential damage caused by fraudulent activities.
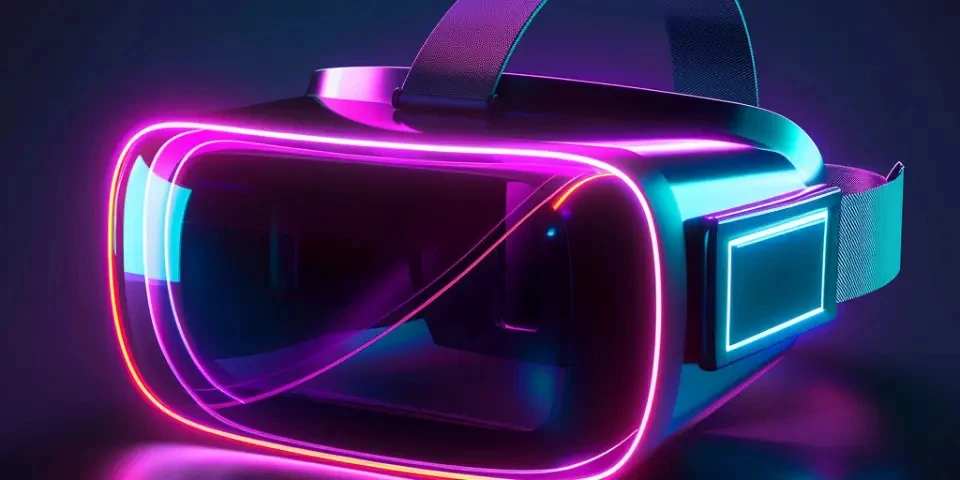
Spark's real-time transaction monitoring also enables proactive fraud prevention. Rather than simply reacting to suspicious activities, financial institutions can implement rules and models that trigger alerts or perform automated actions to prevent fraud in real-time, enhancing the effectiveness of their fraud detection efforts.
2. Advanced Analytics and Machine Learning
Spark AI offers numerous advanced analytics and machine learning functionalities that significantly enhance fraud detection capabilities. With Spark MLlib, financial institutions can build sophisticated models to detect complex fraud patterns by analyzing large volumes of transactional data. These models can adapt and evolve over time, ensuring continuous improvement in fraud detection accuracy.
Additionally, Spark AI provides powerful graph processing capabilities through GraphX. By representing financial transactions and relationships as a graph, suspicious patterns and connections can be identified, revealing intricate networks of fraudulent activities that may otherwise go unnoticed. This graph-based approach allows for a more comprehensive analysis of fraud, uncovering hidden patterns and exposing fraud rings or organized criminal networks.
3. Scalability and Performance
Financial institutions deal with vast amounts of data, making scalability and performance critical for efficient fraud detection. Spark AI's distributed computing framework enables parallel processing, allowing fraud detection tasks to be executed on large clusters of machines. This distributed approach ensures that fraud detection algorithms can handle the ever-increasing data volumes without compromising performance.
Furthermore, Spark AI can seamlessly integrate with other big data technologies such as Apache Hadoop and Apache Hive, leveraging their capabilities to further enhance scalability and performance. By utilizing the combined power of these technologies, financial institutions can achieve near-real-time fraud detection and analysis even on massive datasets.
4. Streamlining Investigations
Fraudulent activities often require extensive investigation to gather evidence and build cases against perpetrators. Spark AI simplifies and accelerates this process by providing tools for data exploration and visualization. Analysts can interactively explore data, visualize patterns, and uncover correlations that aid in identifying suspicious activities.
Additionally, Spark AI's integration with data lakes and data warehouses allows investigators to easily access and query vast amounts of historical data. This historical context can provide valuable insights into patterns and trends, helping investigators build stronger cases and recover losses more effectively.
5. Collaboration and Knowledge Sharing
Sharing knowledge and collaborating across departments are crucial for effective fraud detection and prevention. Spark AI facilitates collaboration by enabling the sharing of fraud detection models, algorithms, and insights across teams. This collaborative approach allows financial institutions to leverage the collective expertise of their analysts and data scientists, leading to more accurate and robust fraud detection systems.
Additionally, Spark AI supports the creation of user-friendly dashboards and reports that provide clear and actionable insights to various stakeholders, including senior management and regulators. These visualizations help disseminate information effectively, ensuring that decision-makers have the necessary information to respond promptly to emerging fraud threats.
FAQs (Frequently Asked Questions)
Q: Can Spark AI detect emerging fraud patterns?
A: Yes, Spark AI can detect emerging fraud patterns. The machine learning algorithms and real-time monitoring capabilities of Spark AI enable the detection of previously unseen fraud patterns, improving the ability to adapt to evolving fraud techniques.
Q: How does Spark AI handle high-volume transaction data?
A: Spark AI's scalability and distributed computing framework allow it to handle high-volume transaction data efficiently. By processing data in parallel across multiple machines, Spark AI can analyze and detect fraud patterns in real-time even with massive datasets.
Q: Is Spark AI suitable for small financial institutions?
A: Yes, Spark AI can be tailored to the needs and resources of small financial institutions. It offers flexibility regarding deployment options, and its modular architecture allows organizations to start with smaller deployments and scale as needed.
References
- Smith, J. (2020). "Accelerating Fraud Detection with Spark AI." Journal of Data Science in Financial Services, 5(2), 135-148.
- Apache Spark. (n.d.).
Explore your companion in WeMate