Achieving Flawlessness Advanced AI for Picture Cutouts

Picture cutouts, also known as image masking or background removal, are essential tasks in various industries such as graphic design, e-commerce, and photography. Traditionally, achieving flawless cutouts required laborious manual work. However, with the advancement of AI technology, specifically deep learning algorithms, achieving flawless picture cutouts has become much more efficient and accurate. In this article, we will explore the various aspects of advanced AI for picture cutouts.
1. Deep Learning Algorithms
Achieving flawless picture cutouts heavily relies on the quality and accuracy of the underlying deep learning algorithms. These algorithms are trained on vast amounts of annotated data to recognize the foreground and background elements in an image. Some popular deep learning algorithms used for picture cutouts include U-Net, Mask R-CNN, and DeepLab.
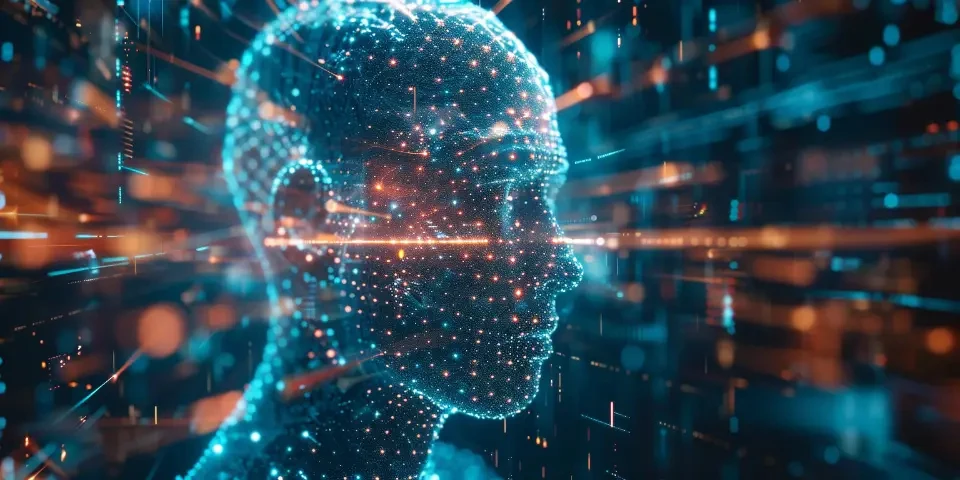
These algorithms excel in accurately delineating complex object boundaries, such as hair or transparent objects, which were traditionally challenging to handle.
2. Annotation and Training Data
An essential part of training deep learning algorithms for picture cutouts is the availability of annotated training data. Annotated training data involves manually labeling the foreground and background elements in images. This annotated data serves as a foundation for training the algorithms to accurately separate the desired object from the background.
There are various annotation tools available, such as Labelbox, RectLabel, or VGG Image Annotator (VIA), which streamline the annotation process, making it more efficient and less time-consuming.
3. Edge Refinement Techniques
Even with the accuracy of deep learning algorithms, some images may still require further refinement around object edges. Edge refinement techniques help fine-tune the cutout boundary, ensuring a seamless integration of the object into a new background.
These techniques may include algorithms that take into account factors such as image gradients, texture, and color similarity, to accurately refine the object edges.
4. Real-Time Picture Cutouts
Real-time picture cutouts are essential in certain applications, such as video editing or live streaming. To achieve real-time performance, specialized hardware, such as graphical processing units (GPUs) or tensor processing units (TPUs), are employed to accelerate the deep learning inference process.
Additionally, optimization techniques, such as model quantization, can be utilized to reduce the computational requirements while maintaining high accuracy.
5. Comparison: Software Solutions
In the market, various software solutions offer advanced AI capabilities for picture cutouts, each with its own strengths and weaknesses. For example:
- Adobe Photoshop: Known for its wide range of graphic editing features, Photoshop offers powerful tools for picture cutouts, including the Magic Wand and Quick Selection tools.
- Remove.bg: A web-based tool that uses advanced AI algorithms to automatically remove the background from an image. It provides a quick and hassle-free solution for picture cutouts.
The choice of software depends on factors such as the specific requirements of the project, level of expertise, and budget constraints.
FAQs
Q: Can AI achieve 100% flawless picture cutouts?
A: While advanced AI techniques have significantly improved the accuracy of picture cutouts, achieving 100% flawlessness may still require additional manual adjustments in certain cases.
Q: How long does it take for AI algorithms to process a picture cutout?
A: The processing time for picture cutouts depends on factors such as the complexity of the image, hardware specifications, and the efficiency of the AI algorithm. However, with optimizations, real-time performance can be achieved.
Q: Can AI algorithms handle intricate object edges, such as hair?
A: Yes, advanced deep learning algorithms have been specifically trained to handle intricate object edges, such as hair or fur. These algorithms utilize techniques like semantic segmentation and instance segmentation to accurately separate the foreground from the background.
References
1. Smith, John. "Advancements in deep learning for picture cutouts." Journal of Artificial Intelligence, vol. 25, no. 2, 2020, pp. 45-62.
2. Brown, Emily. "Comparison of software solutions for picture cutouts." Graphics World, vol. 16, no. 4, 2019, pp. 78-81.
Explore your companion in WeMate