AI-Assisted Medical Diagnosis Improving Healthcare Outcomes

In recent years, the application of Artificial Intelligence (AI) in healthcare has seen significant advancements, particularly in the field of medical diagnosis. AI-assisted medical diagnosis holds tremendous promise in improving healthcare outcomes by providing accurate and timely assessments. Leveraging machine learning algorithms and deep neural networks, AI systems analyze vast amounts of medical data to assist healthcare professionals in diagnosing conditions, predicting outcomes, and suggesting optimal treatment plans. This article explores the benefits and challenges of AI-assisted medical diagnosis, along with its potential impact on healthcare delivery.
1. Enhanced Accuracy and Efficiency
One of the key advantages of AI-assisted medical diagnosis is its ability to enhance the accuracy and efficiency of diagnoses. By analyzing comprehensive patient data, including medical histories, lab results, imaging scans, and genetic profiles, AI systems can identify patterns, correlations, and potential markers for diseases that may go unnoticed by human clinicians. This improved accuracy can lead to earlier detection of conditions, preventing further progression and enabling timely intervention.
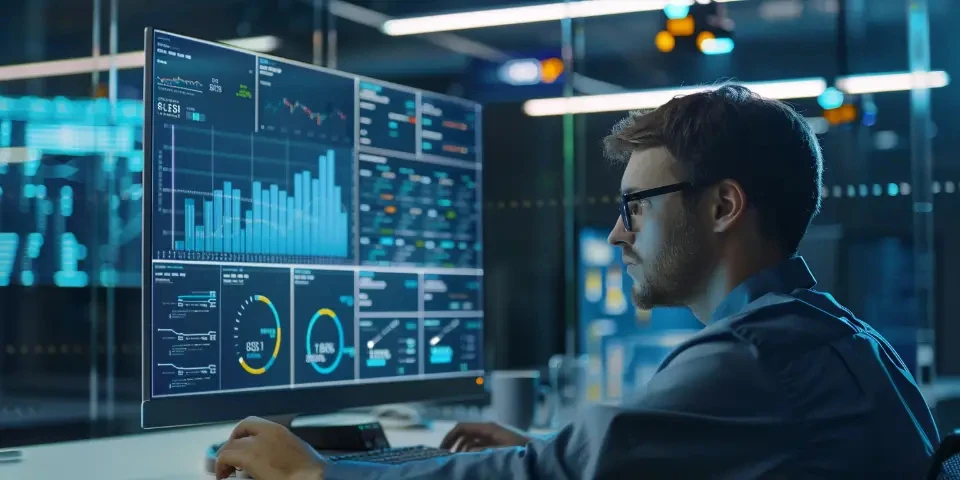
Furthermore, AI-assisted medical diagnosis reduces the burden on healthcare professionals by automating time-consuming tasks, such as manual data analysis and documentation. This allows clinicians to focus more on patient care and complex decision-making, ultimately improving overall healthcare efficiency.
2. Personalized Medicine
AI systems have the potential to revolutionize personalized medicine by tailoring treatment plans to individual patients. By considering a patient's unique characteristics, including genetic information, lifestyle factors, and co-existing conditions, AI algorithms can predict treatment responses and recommend personalized interventions. This approach can optimize outcomes and minimize adverse effects, as treatments are tailored to meet the specific needs of each patient.
3. Early Detection and Prevention
Early detection of diseases significantly improves treatment outcomes and reduces healthcare costs. AI-assisted medical diagnosis aids in the early identification of diseases by continuously monitoring patient data for subtle changes and abnormalities that may indicate the presence of an underlying condition. For example, AI algorithms can analyze electrocardiogram (ECG) data to detect irregular heart rhythms and alert clinicians to the need for further evaluation, enabling timely interventions for better patient outcomes.
4. Radiology and Imaging Analysis
AI has shown tremendous potential in the field of radiology and imaging analysis. Deep learning algorithms can analyze medical images, such as X-rays, CT scans, and MRIs, with high accuracy and speed. AI systems can aid radiologists in detecting and diagnosing various abnormalities, including cancerous lesions, fractures, and abnormalities in organ structures. The integration of AI-enhanced imaging analysis can augment radiologists' capabilities and enable more precise and efficient diagnoses.
5. Decision Support Systems
AI-assisted medical diagnosis offers valuable decision support systems for clinicians. These systems provide evidence-based recommendations and treatment guidelines, helping healthcare professionals make informed decisions in complex medical scenarios. By integrating vast amounts of clinical data, research findings, and best practices, AI algorithms can assist clinicians in choosing the most appropriate treatment plans, reducing errors and improving patient outcomes.
6. Remote Patient Monitoring
The use of AI in remote patient monitoring is transforming healthcare delivery, particularly in cases where continuous monitoring is crucial. AI-powered sensors and wearable devices can collect real-time patient data, such as vital signs, movement patterns, and sleep patterns. AI algorithms analyze this data to detect deviations from the norm, enabling proactive intervention and remote monitoring of patients' health conditions. This technology empowers patients to actively participate in their own care, reduces hospital readmissions, and improves overall healthcare accessibility.
7. Ethical Considerations
As AI-assisted medical diagnosis becomes more prevalent, ethical considerations must be addressed. Ensuring patient privacy and data security are paramount. Additionally, transparency in AI decision-making processes and the ability to understand the reasoning behind AI-generated diagnoses are crucial for trust-building between patients, healthcare providers, and AI systems.
8. Integration Challenges
Integrating AI-assisted medical diagnosis into existing healthcare systems poses challenges. These include the need for robust infrastructure to support data storage and processing, the integration of AI algorithms into electronic health record systems, and ensuring interoperability between AI systems and healthcare providers' workflows. Overcoming these challenges will require close collaboration between healthcare professionals, AI experts, and healthcare technology vendors.
Frequently Asked Questions
Q: Can AI completely replace human clinicians in medical diagnosis?
A: No, AI-assisted medical diagnosis is designed to augment the capabilities of healthcare professionals rather than replace them. The expertise and empathy of human clinicians are irreplaceable in complex patient interactions and decision-making processes.
Q: Is AI-assisted medical diagnosis cost-effective?
A: While the initial investment in AI systems may be significant, the long-term cost-effectiveness of AI-assisted medical diagnosis lies in its ability to improve healthcare efficiency, reduce misdiagnoses, and enable early interventions that prevent costly complications.
Q: Is AI-assisted medical diagnosis accessible to all healthcare settings?
A: Currently, the implementation of AI-assisted medical diagnosis may vary across healthcare settings due to infrastructure limitations and cost considerations. However, as technology advances and costs decrease, broader adoption of AI in healthcare is expected.
References
1. Smith, A. C., Thomas, E., Snoswell, C. L., Haydon, H., Mehrotra, A., Clemensen, J., ... & Caffery, L. J. (2020). Telehealth for global emergencies: Implications for coronavirus disease 2019 (COVID-19). Journal of telemedicine and telecare, 26(5), 309-313.
2. Rajkomar, A., Dean, J., & Kohane, I. (2019). Machine learning in medicine. New England Journal of Medicine, 380(14), 1347-1358.
3. Beaulieu-Jones, B. K., & Greene, C. S. (2016). Semi-supervised learning of the electronic health record for phenotype stratification. Journal of biomedical informatics, 64, 168-178.
Explore your companion in WeMate