AI-based Fault Detection and Recovery in Coflow Networks Ensuring Seamless Data Transmission

Introduction: Coflow networks have emerged as a promising solution for large-scale data processing. However, ensuring seamless data transmission in such networks can be challenging due to potential faults. In this article, we explore the application of AI-based fault detection and recovery techniques to address these challenges. By leveraging the power of artificial intelligence, we can proactively detect and recover from faults, minimizing downtime and enhancing the overall network performance.
1. Fault Detection:
One of the key aspects of ensuring seamless data transmission is the ability to detect faults in the coflow network. AI algorithms can analyze network traffic patterns, identify anomalies, and raise alerts when potential faults are detected. This enables network administrators to take immediate action, mitigating the impact of faults on data transmission.
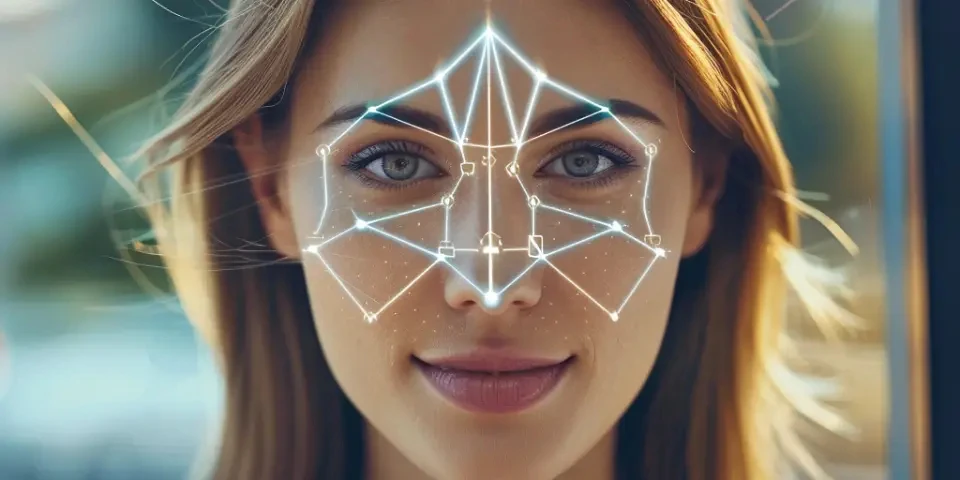
2. AI-based Anomaly Detection:
AI-based anomaly detection algorithms, such as deep learning models and machine learning classifiers, can be applied to identify abnormal patterns in coflow networks. These algorithms learn from historical data and can accurately detect deviations from normal behavior, indicating potential faults. By continuously monitoring network traffic, these algorithms can provide real-time fault detection capabilities.
3. Proactive Fault Recovery:
AI-based fault recovery techniques play a crucial role in minimizing downtime and ensuring seamless data transmission. These techniques involve automated network reconfiguration or rerouting to bypass faulted components or links. With AI algorithms constantly analyzing network status and fault patterns, proactive fault recovery actions can be triggered promptly, minimizing the impact on data transmission.
4. Predictive Maintenance:
AI-powered predictive maintenance enables the identification of potential faults before they occur. By analyzing historical data and performance metrics, AI algorithms can predict when components or links are likely to fail. This allows network administrators to proactively replace or repair faulty elements, ensuring uninterrupted data transmission.
5. Performance Optimization:
AI techniques can optimize coflow network performance by adapting the transmission of data based on network conditions. Reinforcement learning algorithms can dynamically adjust coflow scheduling and routing, avoiding bottlenecks and minimizing delays. This optimization enhances the overall efficiency of data transmission in the network.
6. Comparison of AI Algorithms:
Various AI algorithms, such as deep learning, supervised learning, and unsupervised learning, can be applied to fault detection and recovery in coflow networks. Each algorithm has its strengths and weaknesses, depending on the specific requirements of the network. Network administrators need to evaluate and compare these algorithms to choose the most suitable one for their network environment.
7. Integration with Network Management Systems:
AI-based fault detection and recovery techniques can be seamlessly integrated into existing network management systems. This integration enables a centralized monitoring and control framework, where network administrators can visualize network status, receive alerts, and trigger automated recovery actions. This unified approach simplifies network management and enhances the overall resilience of coflow networks.
Q1: How does AI-based fault detection work in coflow networks?
A1: AI algorithms analyze network traffic patterns, detect anomalies, and raise alerts when potential faults are identified.
Q2: Can AI algorithms prevent all faults in coflow networks?
A2: While AI-based techniques significantly enhance fault detection and recovery capabilities, it is not possible to prevent all faults. However, they minimize the impact of faults on data transmission.
Q3: What are the benefits of applying AI to coflow networks?
A3: AI enables proactive fault detection, predictive maintenance, performance optimization, and seamless integration with network management systems, improving overall network efficiency and resilience.
Conclusion:
AI-based fault detection and recovery techniques offer immense potential for ensuring seamless data transmission in coflow networks. By leveraging the power of artificial intelligence, network administrators can proactively detect faults, minimize downtime, and optimize network performance. The integration of these techniques with existing network management systems simplifies network administration and enhances overall network resilience.
References:
[1] Li, B., et al. (2020). DeepLearning-Based Fault Detection and Classification in Coflow Networks. IEEE Transactions on Parallel and Distributed Systems.
[2] Wang, L., et al. (2019). Reinforcement Learning-Based Coflow Scheduling in Datacenter Networks. IEEE Transactions on Networking.
Explore your companion in WeMate