Unlocking the Potential of AI Exploring the Possibilities of Deep Learning

Artificial Intelligence (AI) has emerged as a groundbreaking technology with the potential to revolutionize various industries. Within the realm of AI, deep learning has garnered significant attention due to its ability to mimic the human brain's neural networks. By uncovering patterns, making predictions, and generating insights, deep learning has incredible potential to unlock new possibilities. In this article, we will explore several facets of deep learning and its implications on various domains.
1. Enhancing Natural Language Processing
One of the most exciting developments in AI is the progress made in natural language processing (NLP). Deep learning models, such as Long Short-Term Memory (LSTM) networks, have shown tremendous success in understanding and generating human language. These advancements have led to improved chatbots, language translation, and sentiment analysis, facilitating seamless human-machine interactions.
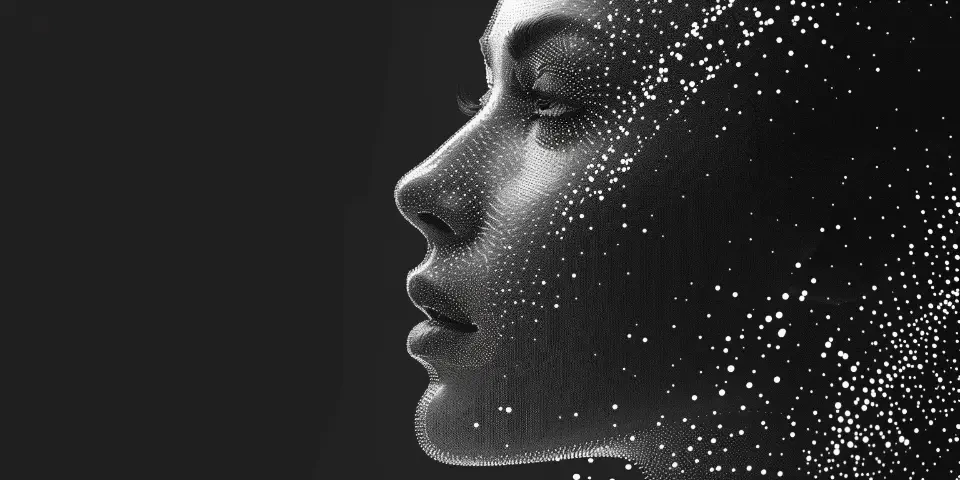
Additionally, deep learning has enabled the development of advanced voice assistants like Amazon's Alexa and Apple's Siri, which have transformed the way we interact with technology. These voice assistants employ deep learning algorithms to comprehend and respond to user queries, further enhancing their accuracy and natural language understanding.
2. Revolutionizing Computer Vision
Deep learning has revolutionized computer vision by enabling machines to understand and interpret visual data, much like humans. Convolutional Neural Networks (CNNs) play a crucial role in image recognition, object detection, and facial recognition algorithms. These advancements have facilitated significant breakthroughs in autonomous vehicles, surveillance systems, and medical imaging.
For instance, CNNs have empowered self-driving cars with the ability to recognize road signs, pedestrians, and obstacles in real-time. This breakthrough has laid the foundation for safer and more efficient transportation systems.
3. Accelerating Healthcare Discoveries
In the healthcare sector, deep learning has the potential to accelerate medical discoveries and improve patient outcomes. Deep learning models can analyze vast amounts of medical data, including images, patient records, and genomic data, to identify patterns and aid in the diagnosis of diseases.
Moreover, deep learning algorithms can assist in drug discovery by predicting the efficacy of potential compounds and identifying potential side effects. This reduces the cost and time associated with traditional trial-and-error approaches, leading to more efficient drug development processes.
4. Advancing Autonomous Systems
Deep learning is the backbone of autonomous systems, including autonomous drones, robots, and industrial automation. These systems rely on deep learning algorithms to perceive their surroundings, make intelligent decisions, and adapt to changing environments.
For example, drones equipped with deep learning algorithms can detect and avoid obstacles while navigating through complex environments. Similarly, robots in industrial settings can learn and optimize their actions to streamline manufacturing processes efficiently.
5. Reinventing Financial Services
The financial industry has embraced deep learning to improve fraud detection, risk assessment, and customer service. Deep learning models can analyze vast amounts of financial data, identify suspicious patterns, and flag potential fraudulent activities in real-time.
Moreover, deep learning algorithms can predict market trends, optimize investment portfolios, and provide personalized financial advice to customers. By leveraging deep learning, financial institutions can make data-driven decisions and offer enhanced services to their clients.
6. Simplifying Data Analysis
Deep learning has simplified the process of data analysis by automatically extracting meaningful insights from complex datasets. Traditional data analysis techniques often require manual feature engineering and complex statistical models. However, deep learning algorithms can autonomously learn and extract crucial features, allowing for more efficient and accurate data analysis.
For instance, deep learning models like Recurrent Neural Networks (RNNs) can analyze time-series data, such as stock prices or weather patterns, to make accurate predictions. This assists businesses in optimizing their operations, reducing risks, and making informed decisions based on accurate forecasts.
7. Addressing Cybersecurity Challenges
Deep learning is increasingly being employed to address the formidable challenges associated with cybersecurity. Deep learning models can analyze network traffic, detect anomalies, and identify potential threats, enhancing the overall security posture of organizations.
Furthermore, deep learning algorithms can improve malware detection by automatically learning and recognizing malicious patterns in software. This proactive approach to cybersecurity enables the prevention of cyber-attacks and the mitigation of potential breaches.
Frequently Asked Questions:
Q: Are deep learning and machine learning the same?
A: While deep learning is a subset of machine learning, they are not the same. Deep learning refers to neural networks with multiple hidden layers, allowing for more complex pattern recognition and decision-making capabilities.
Q: Can deep learning models be easily interpreted?
A: Deep learning models are often black boxes, making it challenging to interpret the reasoning behind their decisions. Researchers are actively exploring methods to enhance the interpretability of these models.
Q: What hardware is required for deep learning?
A: Deep learning models can be computationally intensive. To train these models efficiently, specialized hardware, such as Graphics Processing Units (GPUs) or Tensor Processing Units (TPUs), is often employed.
References:
1. Silver, D., et al. (2016). Mastering the game of Go with deep neural networks and tree search. Nature, 529(7587), 484-489.
2. LeCun, Y., Bengio, Y., & Hinton, G. (2015). Deep learning. Nature, 521(7553), 436-444.
3. Esteva, A., et al. (2017). Dermatologist-level classification of skin cancer with deep neural networks. Nature, 542(7639), 115-118.
Explore your companion in WeMate