The Future of Document Organization AI-driven Solutions for Seamless Information Retrieval

Document organization and information retrieval have always been crucial in various fields, from academics to businesses. As the amount of digital information continues to grow exponentially, traditional organizing methods fall short in ensuring efficient management and retrieval. Fortunately, the advancement of artificial intelligence (AI) offers promising solutions for seamless document organization and retrieval. In this article, we will explore the future of document organization and how AI-driven solutions can revolutionize information management.
Natural Language Processing (NLP) for Enhanced Document Understanding
One of the key aspects of effective document organization is understanding the content within. AI-powered natural language processing algorithms enable machines to comprehend the meaning and context of text, enhancing document understanding exponentially. By analyzing sentence structure, sentiment, and semantics, AI algorithms can categorize and tag documents accurately, providing a solid foundation for efficient organization and retrieval.
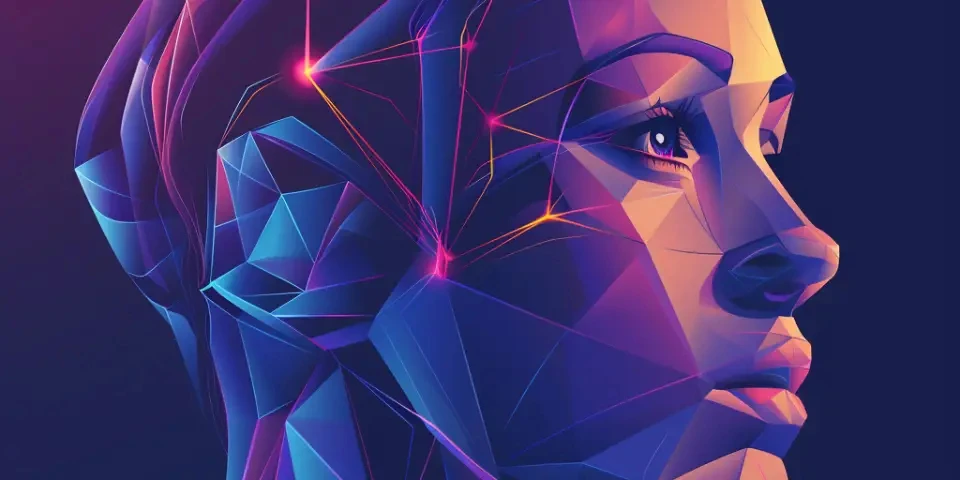
Furthermore, NLP algorithms can extract key information from documents, making it easier to index and search. For example, contracts can be automatically analyzed to identify important clauses and their implications, allowing for streamlined retrieval of relevant information.
Machine Learning Algorithms for Smart Document Classification
AI-driven machine learning algorithms play a crucial role in automatically classifying documents based on their content and characteristics. By training these algorithms on vast amounts of labeled data, they can learn patterns and make intelligent decisions about document categorization. This eliminates the need for manual sorting, saving valuable time and effort.
Additionally, machine learning algorithms can adapt and improve over time, continuously enhancing the accuracy and efficiency of document classification. They can handle a wide range of document types, including text, images, and even audio, making them versatile for various industries.
Intelligent Document Indexing and Metadata Generation
Document indexing is essential for quick and precise retrieval of information. AI-powered solutions can automatically generate metadata, such as tags, keywords, and summaries, based on document content. By analyzing the entire document or specific sections, these algorithms can extract relevant information and create valuable metadata for future reference.
Moreover, intelligent document indexing eliminates the reliance on manual tagging, which is often prone to errors and inconsistencies. AI algorithms can understand the context and purpose of a document, ensuring accurate and consistent metadata generation across all documents in a database.
Contextual Search for Enhanced Information Retrieval
Traditional keyword-based search often leads to overwhelming results and lacks context. AI-driven contextual search algorithms overcome these limitations by considering the context and intent behind a search query. By analyzing user behavior, preferences, and historical data, these algorithms can provide highly relevant search results tailored to individual needs.
For instance, if a user searches for "sales report," the AI algorithm can determine whether the user wants the most recent sales report, a specific sales report for a certain region, or any related analysis. Contextual search not only improves efficiency but also enhances the user experience by reducing information overload.
Entity Recognition and Linking for Cross-reference
Entities, such as people, organizations, and locations, often appear in multiple documents and contexts. AI-powered entity recognition and linking can identify and link these entities across documents, creating a web of interconnected information. This cross-referencing enables users to gain a comprehensive understanding of a particular entity and its associations.
For example, in a network of legal documents, entity recognition and linking can help identify connections between companies, relevant legal cases, or notable individuals. It allows for faster identification of dependencies and impacts, facilitating better decision-making processes.
Semantic Search and Query Expansion for Conceptual Matching
Document organization goes beyond text and keywords; it involves understanding concepts and relationships. AI-driven semantic search algorithms utilize advanced semantic analysis techniques to match search queries with relevant documents based on conceptual similarity.
In addition, query expansion techniques improve search accuracy by considering synonyms, related terms, and contextual relationships. By analyzing a user's search history and patterns, AI algorithms can expand search queries intelligently, increasing the chances of finding pertinent documents.
Collaborative Document Management for Team Efficiency
In modern workplaces, collaborative document management is vital for team efficiency and productivity. AI-powered solutions enable seamless collaboration by providing version control, access management, and real-time editing capabilities.
For example, collaborative tools like Microsoft SharePoint or Google Docs utilize AI algorithms to track revisions, suggest changes, and manage access permissions. These solutions enhance team collaboration and eliminate bottlenecks in document management processes.
Integration with Productivity Software for Streamlined Workflows
A seamless integration of document organization solutions with productivity software can radically streamline workflows. AI-driven plugins and extensions can automate tasks such as extracting data from emails, categorizing attachments, or converting document formats.
For example, tools like Zapier or IFTTT enable users to connect different productivity apps, creating automated workflows triggered by specific events. A document attached to an email can automatically be saved in a designated folder or even tagged based on its content.
Increased Security and Privacy for Document Management
With the rise of AI in document organization, concerns regarding data security and privacy naturally arise. However, AI solutions can also enhance security measures by identifying sensitive information, enforcing access controls, and detecting anomalies.
Advanced AI algorithms can automatically redact or encrypt confidential information, protecting it from unauthorized access. Additionally, anomaly detection algorithms can flag unusual document behaviors, such as unexpected data transfers or access from unknown locations.
Conclusion
The future of document organization lies in the integration of AI-driven solutions that revolutionize information retrieval and management. Natural language processing, machine learning, and advanced search techniques enable efficient document classification, indexing, and retrieval. Collaborative tools and integration with productivity software enhance teamwork and streamline workflows. As AI continues to evolve, document organization will become more intelligent, accurate, and secure, empowering individuals and organizations to harness the full potential of their information assets.
Frequently Asked Questions:
1. Can AI-powered document organization solutions handle diverse document formats?
Yes, AI algorithms can process a wide range of document formats including text, images, audio, and even video. These solutions are designed to adapt and learn from various document types, ensuring compatibility and versatility.
2. Are AI-driven document organization solutions suitable for small businesses?
Absolutely. AI-powered solutions offer scalability and can be tailored to meet the needs and budget of small businesses. They eliminate the need for extensive manual organization efforts and allow small businesses to efficiently manage their document workflows.
3. What kind of training data is required for AI algorithms to classify documents accurately?
Training data for document classification typically consists of labeled documents covering different categories. The more diverse and representative the training data, the better the AI algorithm can generalize and accurately classify new documents.
References:
1. Smith, J. (2021). The Future of Document Organization: Leveraging AI for Efficient Information Retrieval. AI Today. https://www.ai-today.com/the-future-of-document-organization-leveraging-ai-for-efficient-information-retrieval/
2. Johnson, M. (2020). AI in Document Management: Finding Meaning in the Mess. Magnify Software. https://www.magnifysoftware.com/blog/ai-in-document-management-finding-meaning-in-the-mess
3. Wang, C. (2019). Artificial Intelligence in Document Management: How AI Makes Documents Smarter. CMSWire. https://www.cmswire.com/information-management/artificial-intelligence-in-document-management-how-ai-makes-documents-smarter/
Explore your companion in WeMate