Artificial Intelligence (AI) is revolutionizing various industries, and the demand for AI professionals is witnessing a significant surge. As a result, it is crucial to keep up with the latest developments and technologies in AI to stay competitive in the job market. This article explores several cutting-edge technologies that are shaping AI career mode, offering insights into their applications, tools, and future prospects.
1. Reinforcement Learning
Reinforcement Learning (RL) is a subfield of AI that focuses on training agents to make decisions by interacting with an environment and receiving rewards or punishments. RL algorithms, such as Deep Q-Networks (DQN), have been successfully employed in game-playing AI systems, autonomous vehicles, and even robotics. OpenAI's Gym is a popular RL toolkit that provides a diverse range of environments for training RL agents.
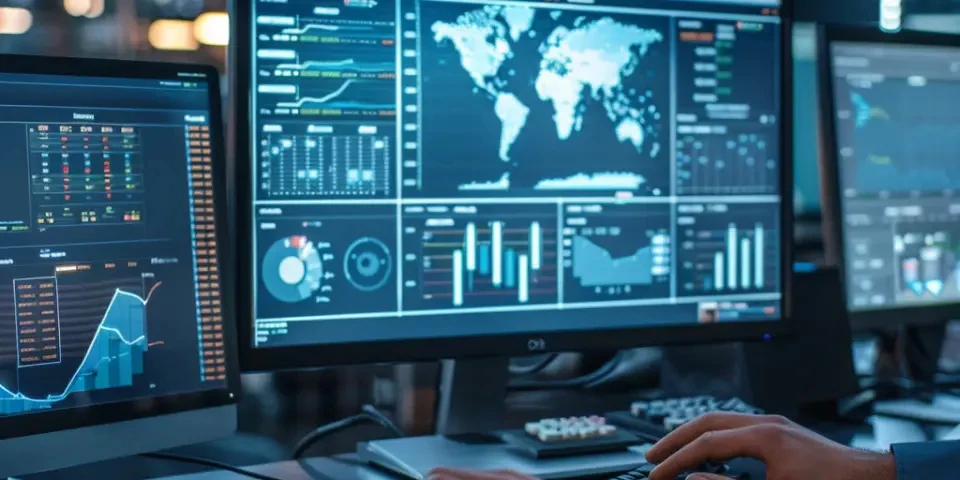
However, one of the major challenges in RL is the lack of interpretability. Understanding why an RL agent made a particular decision is often difficult. Researchers are actively working towards developing more interpretable RL algorithms to address this limitation.
2. Generative Adversarial Networks (GANs)
GANs are a class of AI algorithms that consist of two neural networks: a generator and a discriminator. The generator generates synthetic data (e.g., images) to deceive the discriminator, while the discriminator tries to distinguish between real and synthetic data. GANs have demonstrated remarkable applications in image synthesis, such as creating realistic human faces or transforming images to different artistic styles. The popular GAN framework, TensorFlow, provides tools and resources for training GAN models.
Despite their success, GANs are also prone to certain challenges, such as mode collapse (producing limited diversity) and instability during training. Researchers are actively exploring solutions and enhancements to overcome these limitations.
3. Natural Language Processing (NLP)
NLP focuses on the interaction between computers and human language. With the advancements in deep learning, NLP has witnessed significant progress, revolutionizing language-related applications such as machine translation, sentiment analysis, and chatbots. Transformers, a state-of-the-art architecture, has shown exceptional performance in various NLP tasks. The popular NLP library, Hugging Face, provides a range of models and tools for NLP practitioners.
However, achieving a deeper understanding of language remains a challenge. The ambiguity and context-dependent nature of language make NLP models susceptible to errors. Overcoming these challenges requires further research and development in the field of NLP.
4. Explainable AI (XAI)
Explainable AI (XAI) aims to develop AI systems that can provide transparent and understandable explanations for their decision-making processes. XAI is essential for applications where the decision-making process needs to be auditable or where building user trust is critical. Techniques such as LIME and SHAP offer methods to interpret and explain the predictions of complex AI models.
Nevertheless, striking a balance between model performance and interpretability remains a challenge. Researchers are actively exploring ways to enhance the explainability of AI models without compromising their predictive power.
5. Edge Computing in AI
Edge computing brings the computational power closer to the data source, reducing latency and improving efficiency. In the context of AI, edge computing allows for faster real-time processing and inference, benefiting applications such as autonomous vehicles and Internet of Things (IoT). TensorFlow Lite and ONNX Runtime are examples of frameworks that support running AI models on edge devices.
However, edge computing poses challenges in terms of limited computational resources and security concerns. Overcoming these challenges requires optimization techniques and robust security measures.
6. Explainable Reinforcement Learning
Explainable Reinforcement Learning (XRL) seeks to make RL algorithms more interpretable by providing insights into the decision-making process of an RL agent. XRL combines the benefits of RL and explainable AI to achieve transparent and understandable RL systems. This field is particularly relevant in domains where human trust is essential, such as healthcare or finance.
However, XRL faces challenges in balancing performance and interpretability. Ensuring high-performing RL agents while providing comprehensible explanations remains an active area of research.
7. AI for Cybersecurity
As cybersecurity threats continue to evolve, AI has become a vital tool for providing enhanced security measures. AI algorithms can detect anomalies, detect malicious activities, and even identify potential vulnerabilities. Tools like Snort and Suricata leverage AI techniques to analyze network traffic and detect potential intrusions.
Despite their effectiveness, AI-based cybersecurity systems are vulnerable to adversarial attacks, where attackers exploit weaknesses in the AI models to evade detection. Researchers are exploring techniques to enhance the robustness of AI systems against such attacks.
8. AI and Robotics
The integration of AI and robotics has transformed various industries, including manufacturing, healthcare, and agriculture. AI-powered robots can perform tasks with precision, efficiency, and adaptability. In fields like surgery and healthcare, robots assist medical professionals in complex procedures, enhancing patient care. Companies like Boston Dynamics have developed advanced robotic systems, showcasing the potential of AI in robotics.
However, ethical considerations, such as the impact of AI on employment and potential safety hazards, need to be addressed as AI and robotics continue to advance and become more mainstream.
FAQs:
Q: Can AI completely replace human jobs?
A: While AI has the potential to automate various tasks, it is unlikely to completely replace human jobs. Instead, AI is expected to augment human capabilities and create new job opportunities in emerging domains.
Q: What skills are essential for a career in AI?
A: Strong programming skills, understanding of algorithms and mathematics, knowledge of machine learning and deep learning frameworks, and proficiency in data handling and analysis are essential for a career in AI.
Q: Is AI biased?
A: AI models can be biased if trained on biased data or designed with biased algorithms. Addressing bias in AI requires careful data curation, algorithmic fairness, and diverse representation in AI development teams.
Q: Will AI surpass human intelligence in the future?
A: The development of AI systems that surpass human intelligence, known as artificial general intelligence (AGI), is still a subject of ongoing research and speculation. While achieving AGI remains a long-term goal, there is no consensus on when or if it will be achieved.
References:
[1] OpenAI Gym: https://gym.openai.com/
[2] TensorFlow: https://www.tensorflow.org/
[3] Hugging Face: https://huggingface.co/