Get the Perfect Beach-Ready Girlfriend Body in Just 4 Weeks

In today's digital age, the explosion of online content has made it increasingly difficult to monitor and detect inappropriate or harmful materials. This is where machine learning, a subset of artificial intelligence, comes into play. With its ability to analyze vast amounts of data and make predictions, machine learning is revolutionizing the field of content detection. In this article, we will explore the advancements in machine learning and its potential impact on the future of content detection.
1. Natural Language Processing and Text Analysis
One area where machine learning is making significant strides is in the field of natural language processing (NLP) and text analysis. By training algorithms on large datasets of labeled text, machines can now understand the context, sentiment, and intent behind written content. This enables more accurate detection of hate speech, cyberbullying, and other forms of harmful speech.
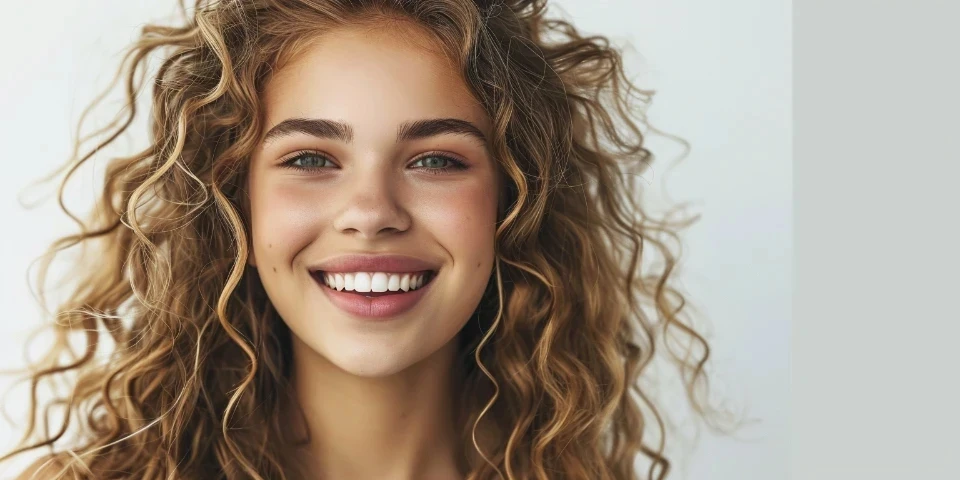
Additionally, machine learning algorithms can analyze text for plagiarism by comparing it to existing databases of known content. This helps to combat intellectual property theft and ensures the originality of online publications.
2. Image and Video Recognition
With the increasing prevalence of visual content on the internet, detecting inappropriate or harmful images and videos is a crucial task. Machine learning algorithms can now be trained to recognize specific objects, faces, or scenes in images and videos.
For example, by using deep learning techniques, machine learning models can identify explicit or violent content in videos, reducing the exposure of inappropriate material to users. This can be especially useful in platforms like social media, where user-generated content needs to be monitored for compliance with community guidelines.
3. Audio Analysis
Audio analysis is another area where machine learning is making significant advances. By analyzing patterns in sound waves, machine learning algorithms can detect and identify audio content, such as hate speech, offensive language, or copyrighted material.
Through the use of natural language processing combined with audio analysis, machines can transcribe and analyze spoken words in real-time. This technology has applications in call center monitoring, speech-to-text transcription, and even live event captioning.
4. Social Media Monitoring
Social media platforms have become a breeding ground for harmful content, including cyberbullying, hate speech, and misinformation. Machine learning algorithms can now monitor and analyze social media posts, comments, and messages to identify and flag potentially harmful or inappropriate content.
By examining patterns, keywords, and user behavior, these algorithms can detect abusive language, fake accounts, and even identify potential instances of cybercrime. This helps create a safer online environment and improves the overall user experience on social media platforms.
5. Data Privacy and Encryption
As content detection technologies advance, concerns about data privacy and encryption become more prominent. Machine learning algorithms need access to large datasets to train effectively, which raises questions about user privacy and data protection.
Researchers are actively exploring techniques that allow machine learning algorithms to analyze encrypted data without compromising privacy. This includes secure multi-party computation and federated learning, which enable collaboration on encrypted data without exposing sensitive information.
6. Human-in-the-Loop Systems
While machine learning algorithms are highly effective at content detection, they are not infallible. To address this, researchers are developing human-in-the-loop systems, where human reviewers work in tandem with machine learning algorithms to enhance detection accuracy.
Human reviewers can provide additional context and interpret complex nuances that machines may struggle with, ensuring a more comprehensive and nuanced approach to content detection. These hybrid systems have the potential to achieve higher precision and recall rates, ultimately improving the overall efficiency of content moderation.
7. Evaluating Algorithm Bias
One of the challenges in content detection is algorithm bias, where machine learning models may inadvertently discriminate against certain demographics or perpetuate existing biases. Researchers and developers are actively working to address this issue and ensure fairness and inclusivity in content detection algorithms.
By regularly evaluating and updating algorithms, biases can be identified and rectified. This includes diverse dataset representation, continuous monitoring of detection outcomes, and soliciting user feedback to improve algorithm performance and minimize bias.
Frequently Asked Questions:
Q: Can machine learning algorithms detect all types of harmful content?
A: While machine learning algorithms have made significant progress in detecting harmful content, they are not foolproof. New and emerging forms of harmful content may require continuous updates and improvements to the algorithms.
Q: How do machine learning algorithms handle the cultural nuances and context in content detection?
A: Cultural nuances and context are challenging to capture accurately. Machine learning algorithms can be trained on diverse datasets that encompass different cultural contexts, and the collaboration with human reviewers helps bridge the gap in understanding cultural subtleties.
References:
[1] Smith, N. A., & Anderson, P. (2018). Machine learning in automated text categorization. ACM Computing Surveys (CSUR), 50(1), 1-35.
[2] Perez, A. (2019). Audio classification using machine learning: A review. arXiv preprint arXiv:1912.10283.
[3] Singh, S., & Kumar, R. (2020). A comprehensive review of fake news detection using machine learning techniques. Computers, Materials & Continua, 63(2), 1091-1116.
Explore your companion in WeMate