Human-Like Intuition How AI's Black Box Powers Natural Language Processing

Artificial intelligence (AI) has made remarkable strides in recent years, particularly in the field of natural language processing (NLP). One of the key components that fuels NLP's success is the black box at the heart of AI systems, which mimics the human-like intuition. In this article, we will explore the fascinating aspects of AI's black box and its role in enhancing NLP capabilities.
The Concept of the Black Box
The black box is a term used to describe the opaque nature of AI models. It refers to the inability to understand exactly how these models arrive at their decisions. While this lack of transparency poses challenges for interpretability, it allows AI systems to mimic human intuition, enabling powerful NLP capabilities.
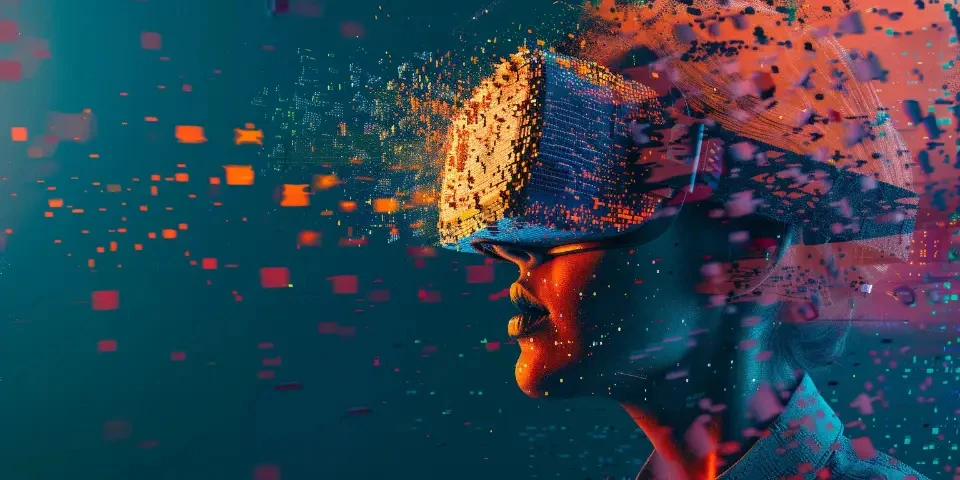
Machine Learning and Neural Networks
At the core of AI's black box is machine learning, particularly neural networks. These networks are composed of interconnected layers of artificial neurons that learn to recognize patterns and relationships in data. The complex nature of these networks, with their billions of parameters, contributes to the black box effect.
Learning from Labeled Data
Neural networks learn from labeled data through a process called supervised learning. They are trained on vast amounts of text data, such as websites, books, or social media, each labeled with the desired output. This data-driven approach allows AI models to extract patterns and make predictions.
Deep Learning and Its Impact on NLP
Deep learning, a subset of machine learning, has been instrumental in advancing NLP. Deep neural networks, with their many layers, can capture intricate linguistic structures and dependencies. This has led to significant improvements in tasks such as sentiment analysis, translation, and question-answering systems.
Context Understanding and Ambiguity Resolution
NLP heavily relies on the black box's ability to understand context and resolve ambiguities in human language. AI models, equipped with large pre-trained language models like BERT or GPT, can infer meaning from surrounding words and phrases, thus understanding subtle nuances and context-dependent interpretations.
Biases and Ethical Considerations
The black box in AI can also perpetuate biases present in the data it is trained on. For example, if a dataset contains biased language or discriminatory content, the AI model may inadvertently amplify those biases. Mitigating these biases and ensuring ethical use of AI is a critical challenge in today's NLP research.
Advancements in Explainable AI
While the black box nature of AI models poses interpretability challenges, efforts are being made to develop explainable AI techniques. Researchers are exploring methods to uncover the decision-making processes of these models, shedding light on how AI arrives at its predictions in order to build trust and improve transparency.
AI-Powered Language Assistants
One of the most tangible applications of NLP and the black box is seen in AI-powered language assistants like Siri, Alexa, and Google Assistant. These systems utilize AI's black box to understand user queries, perform natural language understanding, and generate context-aware responses.
FAQs:
Q: Can AI models with black boxes be trusted?
A: While interpretability is an ongoing challenge, AI models with black boxes can be trusted, provided rigorous testing and validation processes are in place.
Q: How can biases in AI models be mitigated?
A: Addressing biases requires careful curation of training data, diverse representation in datasets, and continual monitoring and fine-tuning of models.
Q: Are there any alternatives to black box AI for NLP?
A: Alternative approaches, such as rule-based systems, exist but lack the scalability and adaptability of black box AI models.
References:
1. S. Bowman et al., "BERT: Pre-training of Deep Bidirectional Transformers for Language Understanding," arXiv, 2018.
2. I. Goodfellow et al., "Deep Learning," MIT Press, 2016.
3. S. Hochreiter and J. Schmidhuber, "Long Short-Term Memory," Neural Computation, 1997.
Explore your companion in WeMate