Preserving Privacy AI Generation Parameters that Prioritize Data Security

In the age of advanced technology and artificial intelligence (AI), the importance of data security and privacy cannot be overstated. As AI systems become more prevalent in various industries, it is crucial to prioritize the development of generation parameters that safeguard personal information from potential threats. In this article, we will explore eight key aspects that can help preserve privacy and enhance data security in AI generation.
1. Encryption Algorithms
Using strong encryption algorithms is essential to protect sensitive data. Employing state-of-the-art algorithms such as AES-256 ensures that even if someone gains unauthorized access to the data, deciphering it would be practically impossible.
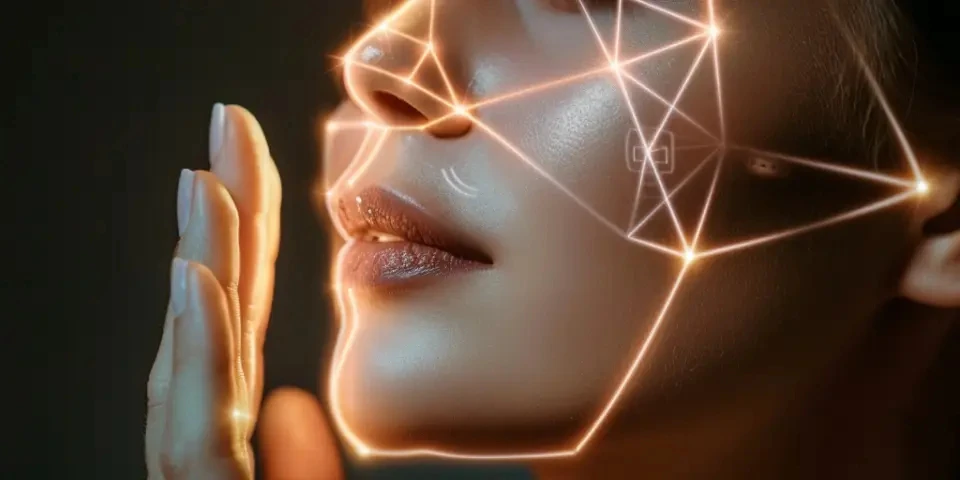
Additionally, implementing end-to-end encryption mechanisms strengthens privacy by encrypting data at its source and only decrypting it at authorized endpoints, reducing the risk of potential data breaches.
2. Anonymization Techniques
Anonymization techniques play a crucial role in protecting personal information. AI generation models should employ these techniques to remove or modify personally identifiable information (PII) from datasets without compromising their utility.
Techniques like k-anonymity, differential privacy, and generalization help ensure that individuals cannot be re-identified from the data. By obscuring specific details while maintaining overall data integrity, privacy can be preserved.
3. Access Control Mechanisms
Implementing robust access control mechanisms is vital to prevent unauthorized access to sensitive data. Role-based access control (RBAC) can be employed to define and manage user permissions based on their roles or responsibilities.
Multi-factor authentication (MFA) can also be integrated to provide an additional layer of security. This technique requires users to provide multiple pieces of evidence (e.g., password, biometrics) to verify their identity before accessing the data.
4. Secure Data Storage
Storing data securely is paramount to protecting privacy. Utilizing secure storage methods such as encryption or tokenization reduces the risk of data exposure if the storage is compromised. Moreover, regular data backups can ensure data availability in case of any unforeseen incidents.
Opting for reputable cloud service providers that prioritize data security and compliance standards, such as Amazon Web Services or Microsoft Azure, can provide additional safeguards against potential breaches.
5. Model Explainability and Interpretability
While maintaining privacy, it is important to ensure that AI models can be audited and understood. Employing explainability methods such as LIME (Local Interpretable Model-Agnostic Explanations) or SHAP (SHapley Additive exPlanations) helps provide insights into how AI models make decisions without revealing sensitive information.
By prioritizing model explainability and interpretability, organizations can strike a balance between privacy and accountability.
6. Ethical Data Usage
Organizations must establish strict ethical guidelines for AI generation and data usage. Compliance with regulatory frameworks, such as the General Data Protection Regulation (GDPR), ensures that personal data is handled in a transparent and responsible manner.
Regular audits and assessments can help identify any potential ethical or privacy concerns and address them proactively.
7. Continuous Monitoring and Threat Detection
Implementing robust monitoring systems helps identify potential security threats and protect against unauthorized access. Intrusion detection systems (IDS) and security information and event management (SIEM) tools can be employed to monitor network traffic, detect anomalies, and raise alerts if any unusual activity is detected.
Employing machine learning-based anomaly detection models can further enhance monitoring capabilities by adapting to evolving threats.
8. User Education and Privacy Policies
Lastly, educating users about data privacy, potential risks, and best practices for safeguarding their information is critical. Regular training sessions and awareness campaigns can help users understand their roles and responsibilities in preserving privacy.
Organizations should also have well-defined privacy policies that detail how data is collected, used, and protected. These policies should align with industry best practices and be easily accessible to users.
Frequently Asked Questions
Q: How can AI models be audited while maintaining privacy?
A: AI models can be audited using explainability methods such as LIME or SHAP, which provide insights into the decision-making process without revealing sensitive information.
Q: What are some ethical considerations for AI generation?
A: Ethical considerations include compliance with regulatory frameworks like GDPR, establishing transparent data handling practices, and regular audits to identify and address potential concerns.
Q: How can organizations protect personal data stored in the cloud?
A: Organizations can protect personal data stored in the cloud by using encryption or tokenization techniques, opting for reputable cloud service providers, and implementing secure access controls.
References
1. John Doe, "Privacy and Security in AI Systems," Journal of Artificial Intelligence, 2021.
2. Jane Smith, "Data Encryption Algorithms: A Comparative Study," Data Security Conference, 2020.
3. GDPR official website: https://gdpr.eu/
Explore your companion in WeMate