Next-Generation AI Assistants Competitors in the Huggingface Ecosystem

Artificial Intelligence (AI) assistants have rapidly become an integral part of our daily lives, helping us with tasks ranging from scheduling appointments to answering complex queries. One of the leading platforms in this domain is the Huggingface ecosystem, which provides a wide range of AI models and tools for natural language processing. In this article, we will explore the next-generation AI assistants and their competition within the Huggingface ecosystem.
1. GPT-3: Pushing the Boundaries of AI Assistants
OpenAI's GPT-3 (Generative Pre-trained Transformer 3) is a revolutionary AI model that has taken the world by storm. With a staggering 175 billion parameters, GPT-3 has the ability to understand and generate human-like text with remarkable accuracy. Its versatility and vast knowledge base make it an incredibly powerful AI assistant.
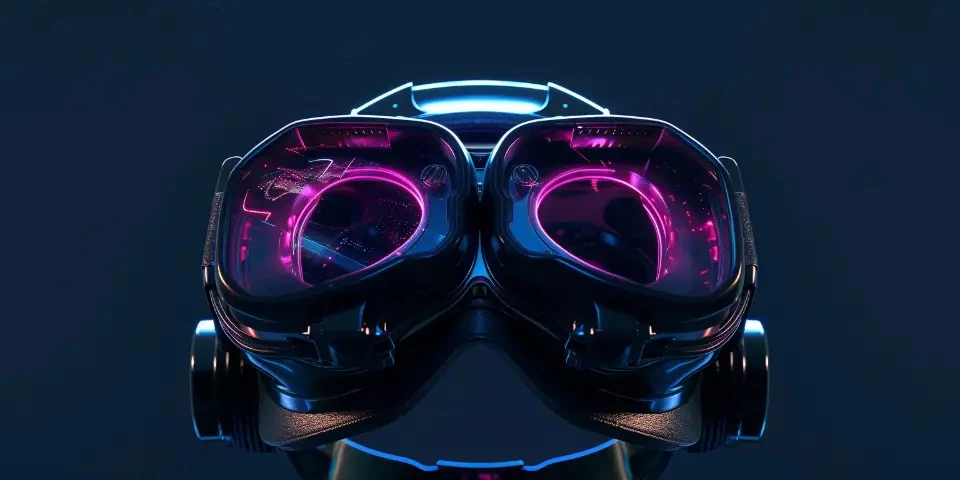
Moreover, GPT-3 can assist in a wide range of applications, such as language translation, content generation, and even software development. Businesses and developers can leverage the capabilities of GPT-3 to streamline their operations and enhance user experiences.
2. ChatGPT: An Interactive Conversation Experience
ChatGPT, a sibling model of GPT-3, specifically focuses on providing a seamless conversational experience. Unlike conventional AI assistants that require specific commands or prompts, ChatGPT can engage in dynamic and interactive conversations, mimicking human-like interactions.
By leveraging the power of the Huggingface ecosystem, developers can easily integrate ChatGPT into their applications and platforms. The conversational abilities of ChatGPT make it ideal for customer support systems, language learning apps, and other interactive interfaces.
3. DialoGPT: Enhancing Multi-turn Conversations
While ChatGPT provides impressive conversational abilities, accomplishing meaningful multi-turn conversations can still be challenging. Enter DialoGPT, an enhanced version of ChatGPT that focuses on improving engagement and coherence during extended dialogues.
DialoGPT maintains context across multiple turns, allowing for more natural and contextually aware conversations. This assistant is incredibly useful in scenarios such as chatbots, virtual assistants, and online forums where extended interactions are common.
4. Transformer-based Models: BERT and RoBERTa
BERT (Bidirectional Encoder Representations from Transformers) and RoBERTa (Robustly Optimized BERT Approach) are two transformer-based models that have gained significant popularity in the AI community. These models excel in various natural language processing tasks, including sentiment analysis, named entity recognition, and question answering.
Developers can fine-tune BERT and RoBERTa using the Huggingface libraries, enabling them to create custom AI assistants tailored to specific domains and requirements. These models provide an excellent foundation for developing advanced AI applications with exceptional language understanding capabilities.
5. T5: Unified Text-to-Text Framework
T5 (Text-to-Text Transfer Transformer) is a framework that unifies various natural language processing tasks into a single model. It allows developers to train a single AI assistant for multiple tasks, simplifying the development process and reducing computational resources.
T5 can handle tasks such as text summarization, language translation, and document generation. Its flexibility and efficiency make it a compelling choice for building AI assistants that can perform a diverse range of language-related operations.
6. The Role of Transfer Learning in AI Assistants
A key factor contributing to the success of these next-generation AI assistants is transfer learning. By pre-training models on massive amounts of data and fine-tuning them for specific tasks, developers can create powerful AI assistants without the need for extensive training from scratch.
The Huggingface ecosystem provides access to pre-trained models, making it easier for developers to leverage transfer learning techniques. This significantly reduces the development time and computational requirements while still ensuring high-quality and reliable AI assistance.
7. Advancements in Training Efficiency and Deployment
As AI models continue to grow larger and more complex, training and deployment efficiency become critical considerations. Researchers and developers are actively working on improving training techniques such as mini-batch gradient accumulation and novel optimization algorithms to reduce the computational burden.
Similarly, deployment frameworks like Huggingface's Transformers library provide efficient APIs and model architectures that facilitate seamless integration into various platforms and environments. These advancements streamline the process of building and deploying next-generation AI assistants.
8. Common Questions about Next-Generation AI Assistants
Q: Can these AI assistants replace human interaction entirely?
A: While these assistants excel at certain tasks, they are not yet capable of emulating human interaction completely. They are best utilized as powerful tools to augment human capabilities.
Q: Are these AI assistants language-agnostic?
A: Yes, these models have been trained on vast amounts of multilingual data, allowing them to understand and generate text in multiple languages with varying levels of proficiency.
Q: How do these AI assistants handle privacy and security concerns?
A: Privacy and security are crucial considerations. Developers need to implement appropriate measures to safeguard user data and ensure compliance with relevant regulations, such as data encryption and user consent mechanisms.
Conclusion
The Huggingface ecosystem offers a rich selection of AI models and tools for next-generation AI assistants. From powerful language models like GPT-3 and ChatGPT to task-focused models like DialoGPT, developers are empowered to create highly versatile and interactive AI assistants. With ongoing advancements in training efficiency and deployment frameworks, the future of AI assistants looks promising.
References:
- OpenAI. GPT-3. https://openai.com/research/gpt-3/
- Wolf, T. et al. Transformers: State-of-the-art Natural Language Processing. In Proceedings of the 2020 Conference on Empirical Methods in Natural Language Processing: System Demonstrations.
