Next-Level Recommendations How Vespa Search Utilizes AI to Deliver Tailored Suggestions

In today's digital world, personalized recommendations play a crucial role in enhancing user experiences across various platforms. Whether it's suggesting products, articles, or even friends, the ability to deliver tailored suggestions can significantly impact engagement and satisfaction. Vespa Search, powered by advanced Artificial Intelligence (AI) algorithms, is one such platform that takes recommendations to the next level. In this article, we will explore how Vespa Search utilizes AI to deliver personalized suggestions, revolutionizing the way we discover and engage with content.
1. Understanding User Preferences
At the core of Vespa Search's recommendation engine lies a sophisticated AI model that analyzes user behavior, preferences, and historical data. By leveraging advanced machine learning techniques, Vespa Search can accurately understand each user's individual preferences, allowing for highly personalized recommendations.
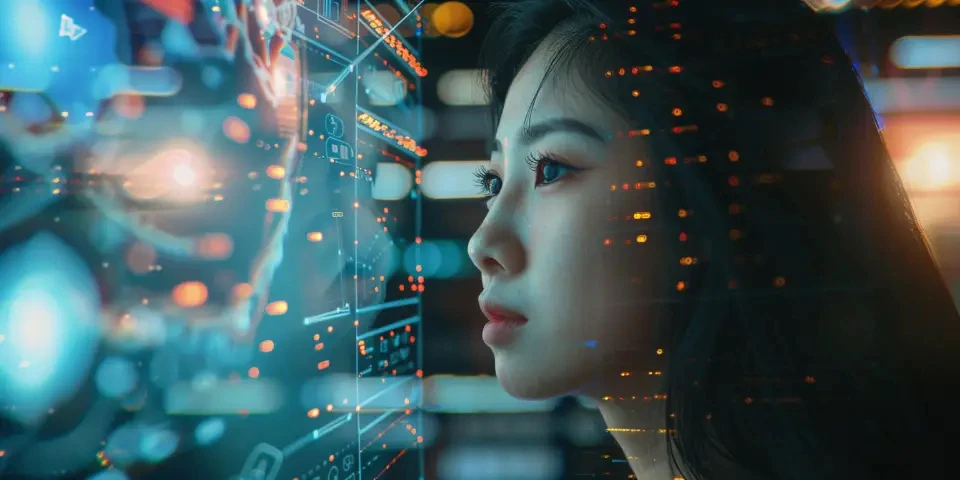
Moreover, Vespa Search continually updates its user profiles based on real-time interactions, enabling the system to adapt and provide up-to-date suggestions. This dynamic approach ensures that recommendations remain relevant even as user preferences evolve over time.
2. Context-Aware Recommendations
Vespa Search creates context-aware recommendations by considering various factors such as location, time, and device type. By incorporating these contextual attributes into the recommendation algorithm, Vespa Search can deliver suggestions that are perfectly tailored to the user's current situation.
For example, if a user is searching for restaurants on their mobile device while in a specific city, Vespa Search can provide recommendations based on the user's location, preferences, and cuisines popular in that area. This level of personalization enhances the user experience by offering relevant suggestions in real-time.
3. Collaborative Filtering
Vespa Search incorporates collaborative filtering techniques to increase the accuracy of its recommendations. Collaborative filtering uses data from multiple users to predict the preferences of an individual based on their similarity to others.
By analyzing patterns in user behavior and identifying users with similar tastes, Vespa Search can recommend items that users with similar preferences have enjoyed. This approach not only enhances the quality of recommendations but also helps users discover new and relevant content outside their immediate circle.
4. Content-Based Filtering
In addition to collaborative filtering, Vespa Search utilizes content-based filtering to deliver personalized suggestions. Content-based filtering takes into account the attributes and characteristics of the items themselves, rather than relying solely on user behavior.
For example, if a user frequently searches for and reads articles related to technology, Vespa Search's content-based filtering algorithm will recommend other technology-focused articles that share similar keywords, topics, or themes. This method enables Vespa Search to deliver recommendations that align with the user's specific interests, improving overall engagement.
5. Serendipity: Recommendations beyond Predefined Preferences
Vespa Search goes beyond traditional recommendation approaches by incorporating a serendipity factor into its algorithm. Serendipity refers to the discovery of pleasant surprises or unexpected recommendations that may lie outside a user's predefined preferences.
By occasionally suggesting content that deviates from a user's typical interests but aligns with their overall profile, Vespa Search introduces users to new and exciting possibilities. This emphasis on serendipity encourages exploration, expanding users' horizons and fostering a sense of discovery.
6. Real-Time Feedback Loop
Vespa Search maintains a real-time feedback loop that allows users to provide explicit feedback on recommendations. This feedback loop helps refine the AI models and algorithms, enhancing the accuracy and relevancy of future recommendations.
Users can rate and provide feedback on the suggested items, indicating whether they found them relevant or valuable. Vespa Search then utilizes this feedback to continuously fine-tune its recommendations, ensuring an ever-improving user experience.
7. Privacy and Data Security
Privacy and data security are paramount when it comes to utilizing user data for tailored recommendations. Vespa Search prioritizes user privacy by anonymizing and securely handling user information.
Although Vespa Search collects and analyzes data to generate personalized recommendations, it does so while adhering to rigorous privacy standards. Users can have confidence in Vespa Search's commitment to protecting their personal information and ensuring data security.
8. Integration with Third-Party Platforms
Vespa Search aims to integrate seamlessly with third-party platforms, enabling businesses to leverage its AI-powered recommendation engine. By integrating Vespa Search, platform owners can provide their users with highly tailored, personalized recommendations, enhancing the overall user experience and increasing engagement.
Whether it's e-commerce platforms, news websites, or social media networks, Vespa Search can seamlessly integrate and deliver recommendations that align with the specific needs and preferences of each platform's users.
Overall, Vespa Search's implementation of AI and advanced recommendation algorithms sets it apart from traditional recommendation systems. By combining user preferences, contextual information, collaborative filtering, content-based filtering, serendipity, and a real-time feedback loop, Vespa Search delivers next-level personalized recommendations that revolutionize the way we discover and engage with content.
Frequently Asked Questions
Q: How does Vespa Search ensure privacy while utilizing user data?
A: Vespa Search prioritizes user privacy and employs strict measures to anonymize and securely handle user information. The platform adheres to rigorous privacy standards to protect personal data.
Q: Can Vespa Search recommend items outside a user's predefined preferences?
A: Yes, Vespa Search incorporates a serendipity factor into its recommendation algorithm, occasionally suggesting content that may lie outside a user's typical interests but aligns with their overall profile. This approach encourages users to explore and discover new possibilities.
Q: How does Vespa Search adapt to evolving user preferences?
A: Vespa Search continually updates user profiles based on real-time interactions. This dynamic approach ensures that recommendations remain relevant even as user preferences evolve over time.
References
1. Vespa Search Official Website:
2. "The role of serendipity in recommender systems" - User Modeling and User-Adapted Interaction Journal
3. "Privacy-preserving collaborative filtering" - Proceedings of the ACM SIGKDD International Conference on Knowledge Discovery and Data Mining
