The rapid advancements in Artificial Intelligence (AI) have revolutionized various industries and transformed the way we live and work. However, one challenge that often arises in AI applications is the stability of diffusion. In this article, we will explore the potential of stable diffusion in AI applications and how it can be unlocked to enhance performance and reliability.
1. Understanding Stable Diffusion
Stable diffusion refers to the ability of an AI system to maintain consistent and reliable performance over time, regardless of changing circumstances or inputs. It ensures that the AI model doesn't suffer from catastrophic forgetting or performance degradation when exposed to new or previously encountered data.
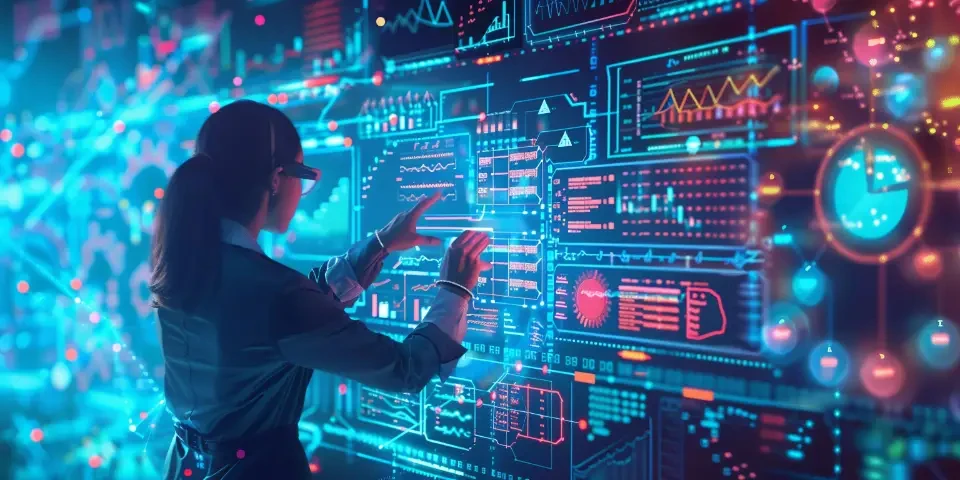
2. Addressing Catastrophic Forgetting
Catastrophic forgetting occurs when an AI model loses previously learned knowledge when new data is introduced. Stable diffusion techniques, such as regularization and continual learning, can effectively mitigate this issue, allowing AI models to retain and build upon past knowledge while accommodating new information.
3. Improving Adaptability in Dynamic Environments
In dynamic environments where data distributions change over time, stable diffusion enables AI models to adapt and generalize their knowledge effectively. Techniques like online learning and reinforcement learning with exploration strategies facilitate continuous learning and adaptation in such scenarios.
4. Enhancing Robustness in Noisy Data
In real-world applications, AI models often encounter noisy data, which can impact their performance and accuracy. Stable diffusion techniques, including robust optimization and data augmentation, can help AI models maintain stable performance in the presence of noise, improving their reliability and usability.
5. Expanding AI Applications in Unseen Domains
Stable diffusion plays a crucial role in enabling AI models to work effectively in unseen or unfamiliar domains. Through transfer learning and domain adaptation techniques, AI models can leverage prior knowledge from related domains and quickly adapt to new tasks or environments.
6. Overcoming Data Scarce Scenarios
In scenarios where labeled data is limited, stable diffusion techniques such as semi-supervised learning and active learning can be employed. These techniques intelligently select and utilize information from unlabeled data to enhance model performance and bridge the gap caused by data scarcity.
7. Ensuring Ethical and Fair AI
Stable diffusion also promotes the development of ethical and fair AI systems. By continuously learning from diverse data sources and incorporating fairness-aware algorithms, AI models can mitigate bias and ensure equitable outcomes across different demographic groups.
8. Combining Stable Diffusion with Explainability
Explainable AI is gaining traction to improve transparency and trust in AI systems. Integrating stable diffusion with explainability techniques, such as attention mechanisms and counterfactual explanations, can provide insights into how the AI model is learning and making predictions, further enhancing its reliability and interpretability.
Frequently Asked Questions:
1. How does stable diffusion address performance degradation?
Stable diffusion techniques, such as regularization and continual learning, prevent catastrophic forgetting and ensure consistent performance by retaining past knowledge while accommodating new information.
2. Can stable diffusion be applied to any AI model?
Yes, stable diffusion techniques can be applied to various AI models, including neural networks, decision trees, and support vector machines. The specific techniques and approaches may vary depending on the model architecture.
3. How does stable diffusion handle noise in data?
Stable diffusion techniques, such as robust optimization and data augmentation, help AI models maintain stable performance in the presence of noisy data by reducing the impact of noise or generating additional training examples.
References:
1. DeepMind. (2020). Continual Learning. https://deepmind.com/learning-resources/continual-learning
2. Silver, D., et al. (2013). Online learning and stochastic optimization. Cambridge University Press.
3. Goodfellow, I., et al. (2014). Transfer learning. In International Conference on Learning Representations (ICLR).