Reliable AI Solutions Unlocking the Potential of Stable AI Apps

Artificial Intelligence (AI) has emerged as a game-changing technology across various industries, revolutionizing the way companies operate and improving efficiency. However, the reliability of AI solutions has been a concern for many businesses. Ensuring stable AI applications is essential to unlock the full potential of this technology. In this article, we will explore the key aspects of reliable AI solutions and how they can be achieved.
1. Robust Data Management
Reliable AI solutions require a strong foundation of clean and quality data. Data management practices must include data cleaning, normalization, and feature selection to ensure accurate and reliable predictions. Implementing proper data governance, such as data validation and verification processes, helps in maintaining data integrity.
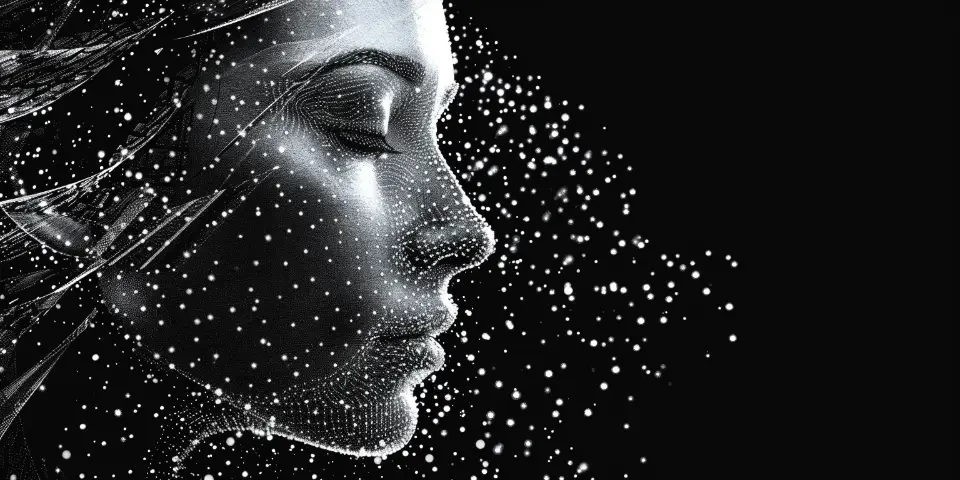
2. Effective Model Training
The training process plays a crucial role in the reliability of AI applications. Using diverse and representative datasets for training models reduces biases and improves the generalizability of the AI system. Regular retraining and fine-tuning of models based on updated data ensure that the AI app stays reliable over time.
3. Ethical Considerations
Reliability in AI solutions goes beyond technical aspects. Ethical considerations should be integrated into the development process to ensure fair and unbiased outcomes. Transparent algorithms, explainable AI techniques, and ethical guidelines protect against discrimination and potential pitfalls.
4. Model Interpretability
Interpretable AI models enable users to understand and trust the decisions made by the system. Techniques such as LIME (Locally Interpretable Model-Agnostic Explanations) and SHAP (SHapley Additive exPlanations) provide insights into how AI models arrive at their predictions, enhancing trustworthiness.
5. Continuous Monitoring
A reliable AI solution requires constant monitoring to identify and address issues promptly. Implementing real-time monitoring and logging mechanisms allows for quick detection of anomalies or performance degradation. It also enables proactive maintenance and fine-tuning of the AI system.
6. Robust Security Measures
AI solutions often deal with sensitive data, making security a crucial aspect of reliability. Implementing encryption, access controls, and secure communication protocols ensures the protection of data and prevents unauthorized access or manipulation.
7. User Feedback Integration
Users' feedback is invaluable for improving the reliability of AI applications. Collecting and analyzing user feedback helps identify areas for improvement, optimize algorithms, and enhance the user experience. Regular user surveys or feedback loops can be implemented.
8. Expert Collaboration
Collaboration with domain experts is essential for developing reliable AI solutions. Experts provide valuable insights into the data, domain-specific challenges, and potential biases. Their involvement ensures the AI app aligns with real-world requirements and improves reliability.
9. Testing and Validation
Thorough testing and validation are critical to ensure the reliability of AI applications. Rigorous testing methodologies, including unit testing, functional testing, and stress testing, help identify and address any issues or vulnerabilities before deployment.
10. Compliance with Regulations
Adhering to legal and regulatory requirements is crucial for reliable AI apps. Compliance with data protection laws, such as GDPR, and industry-specific regulations ensures proper handling of data, maintaining trust and reliability.
11. Benchmarking and Comparison
Benchmarking AI solutions against industry standards and competing products helps understand the reliability of the system. Comparative analysis and performance evaluation provide insights into the strengths and weaknesses of the AI app, aiding improvement efforts.
12. Scalability and Performance
AI solutions should be designed for scalability and high performance to handle increasing data volumes and user demands. Utilizing distributed computing, cloud-based infrastructure, or scalable AI platforms ensures reliability even under heavy loads.
13. Regular Updates and Maintenance
Continual updates and maintenance are necessary to keep AI solutions reliable. Patching vulnerabilities, incorporating new features, and staying updated with the latest technologies guarantees a stable and secure AI app.
14. Integration with Human Intelligence
Combining AI capabilities with human expertise can enhance reliability. Human-in-the-loop approaches, where AI suggestions are evaluated by humans, help prevent errors and improve the overall accuracy and reliability of AI applications.
15. Addressing Bias and Fairness
AI solutions must address bias and fairness concerns to ensure reliability. Regular audits, bias detection algorithms, and diverse training data help avoid biased decisions and maintain the equitable performance of AI apps across different groups.
Frequently Asked Questions (FAQs)
Q: How can businesses ensure the reliability of AI solutions?
A: Businesses can ensure reliability through robust data management, effective model training, ethical considerations, and continuous monitoring, among other key aspects.
Q: Why is model interpretability important for reliable AI?
A: Model interpretability helps users understand and trust the AI system's decisions, enhancing its reliability and transparency.
Q: How can user feedback contribute to the reliability of AI applications?
A: User feedback provides insights for improvement, algorithm optimization, and enhancing the overall user experience, thereby increasing the reliability of AI apps.
