Shaky Privacy Measures Exploring the Unstable Security of AI Systems

In recent years, artificial intelligence (AI) systems have become increasingly integrated into our everyday lives, revolutionizing various sectors such as healthcare, finance, and transportation. However, with the rapid advancement of AI technology, concerns regarding the privacy and security of these systems have also emerged. This article will delve into the unstable security of AI systems, highlighting several aspects that make their privacy measures shaky.
Inadequate Data Protection
One of the fundamental issues with AI systems lies in the inadequate protection of data, which forms the backbone of these technologies. The sheer volume of data processed by AI systems presents numerous challenges in terms of privacy. Organizations may struggle to effectively anonymize sensitive data, leaving individuals at risk of identification. Additionally, there is a lack of standardized encryption protocols, making it easier for hackers to intercept or manipulate data in transit.
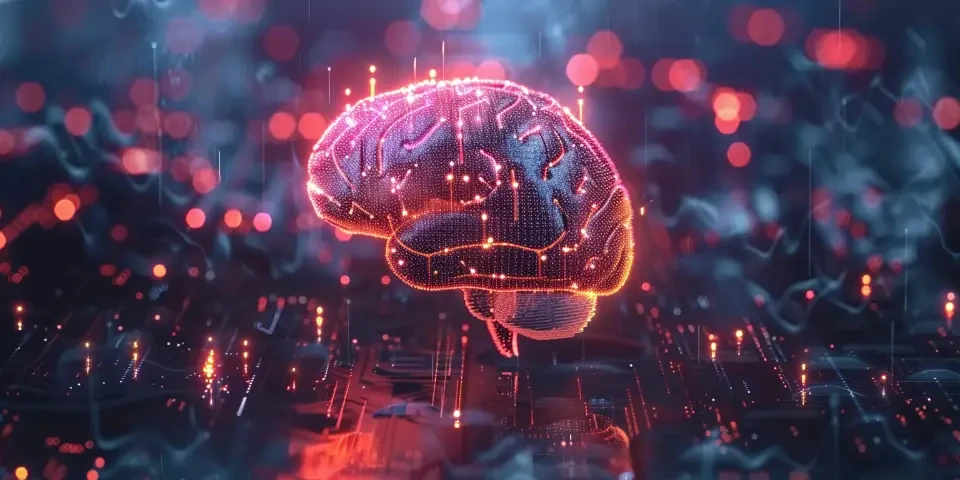
Furthermore, the increasing reliance on third-party data sources introduces uncertainties in data privacy. AI systems often rely on large datasets provided by external entities, which may not have stringent privacy policies in place. As a result, the risk of data breaches and unauthorized access to personal information becomes a prevalent concern.
Biased Algorithmic Decision-Making
Another critical aspect of AI system security is the potential for biased algorithmic decision-making. AI systems are trained on vast amounts of historical data, which may contain inherent biases. These biases can be inadvertently amplified by the algorithms, leading to discriminatory outcomes in various domains such as hiring practices and criminal justice.
Moreover, the opacity of some AI algorithms poses challenges in identifying and rectifying biases. Complex machine learning models often make decisions without providing clear explanations, making it difficult for individuals to challenge or understand the outcomes. This lack of transparency creates an additional layer of vulnerability in the overall security of AI systems.
Insufficient Regulation and Compliance
The current regulatory landscape concerning AI privacy and security is often seen as inadequate. The rapid pace at which AI technology evolves surpasses the ability of legislation to keep up. As a result, there are limited legal guidelines specifically tailored to address the unique challenges posed by AI systems.
Furthermore, compliance with existing privacy regulations such as the General Data Protection Regulation (GDPR) can be difficult to enforce in the context of AI. The complexity of AI systems makes it harder to determine accountability and responsibility for potential breaches. This ambiguity ultimately weakens the security and privacy measures implemented.
Integrity of Training Data
The integrity of training data is crucial for the robustness and security of AI systems. However, ensuring the accuracy and reliability of training data presents significant challenges. Data poisoning attacks have gained attention in recent years, where adversarial actors inject malicious data into the training set to manipulate AI system behavior.
Moreover, the potential for inadvertent inclusion of biased or unrepresentative training data further undermines the security of AI systems. Without careful curation and verification of training data, AI algorithms may learn from flawed or misleading information, resulting in compromised security and privacy.
Emerging Threats in Adversarial Attacks
Adversarial attacks pose a significant threat to the security of AI systems. These attacks aim to manipulate the behavior of AI models by adding imperceptible perturbations to input data, leading to incorrect outputs. For instance, an adversarial attack on an image recognition system could cause it to misclassify a stop sign as a speed limit sign.
As AI algorithms become more widely deployed, the potential impact of adversarial attacks increases. The constantly evolving nature of these attacks makes it challenging to develop robust defense mechanisms. This instability in security measures exposes AI systems to vulnerabilities, further compromising privacy.
Insider Threats and Data Access
Insider threats represent a considerable risk to the security and privacy of AI systems. Individuals with authorized access to AI systems and their underlying datasets may intentionally or unintentionally misuse or compromise sensitive information. Whether it is a disgruntled employee or an accidental mishap, the consequences can be severe.
Implementing robust user access controls and regularly auditing data access logs are key measures to mitigate insider threats. However, maintaining a comprehensive security framework to prevent unauthorized data access remains a significant challenge, particularly when dealing with large-scale AI systems.
Challenges in Explainability and Auditing
Explainability and auditing of AI systems are essential for ensuring security and privacy. Yet, many AI algorithms operate as black boxes, lacking transparency in their decision-making processes. This lack of interpretability hinders effective auditing and the ability to identify potential vulnerabilities or malicious intent.
Developing explainable AI models and establishing comprehensive auditing mechanisms are critical steps in strengthening the security of AI systems. Techniques such as rule-based explanations and algorithmic transparency frameworks can enable a deeper understanding of AI system behavior and aid in identifying potential privacy risks.
Conclusion
The security and privacy of AI systems remain a pressing concern, given their increasing integration into various aspects of our lives. Inadequate data protection, biased algorithmic decision-making, insufficient regulation, and compliance, integrity of training data, emerging adversarial attacks, insider threats, and challenges in explainability and auditing all contribute to the shaky privacy measures implemented in AI systems.
Addressing these issues necessitates collaboration between researchers, policymakers, and industry experts to establish robust privacy policies, stringent regulations, and secure technical frameworks. By proactively addressing the challenges and vulnerabilities, we can pave the way for trustworthy AI systems that prioritize security and privacy.
Frequently Asked Questions
Q: Can AI systems be completely secure and protect privacy?
A: Achieving complete security in AI systems is challenging due to the constantly evolving nature of threats and vulnerabilities. However, by implementing rigorous security measures, regularly updating defenses, and adhering to privacy regulations, a higher level of security and privacy can be achieved.
Q: How can individuals protect their privacy while using AI systems?
A: Individuals can take steps to protect their privacy while using AI systems by reading privacy policies, limiting the amount of personal information shared, regularly updating passwords, and being mindful of the types of data shared with AI applications.
Q: What are some potential consequences of compromised AI system security?
A: Compromised AI system security can lead to various consequences, such as unauthorized access to personal information, misuse of sensitive data, biased decision-making, and the potential for cyberattacks.
References
[1] Allen, G. et al. (2020) Toward Trustworthy AI Development: Mechanisms for Supporting Verifiable Claims. arXiv:2004.07213.
[2] Mittelstadt, C. (2019) AI in Society: Privacy, Fairness, and Transparency. International Journal of Data Science and Analytics, 8(4), 279-286.
[3] Liu, Y. et al. (2021) Investigating Privacy Leakage of Deep Neural Networks by Membership Inference. arXiv:2101.09332.
Explore your companion in WeMate