Stay Ahead of the Competition How AI Can Predict Future Market Trends

In today's rapidly changing business landscape, staying ahead of the competition is crucial for success. One way to gain a competitive edge is by leveraging artificial intelligence (AI) to predict future market trends. AI has the potential to revolutionize the way businesses operate, enabling them to make informed strategic decisions and adapt to dynamic market conditions. This article explores the various ways in which AI can be used to predict future market trends and offers insights into the benefits and challenges of implementing AI-driven predictive analytics.
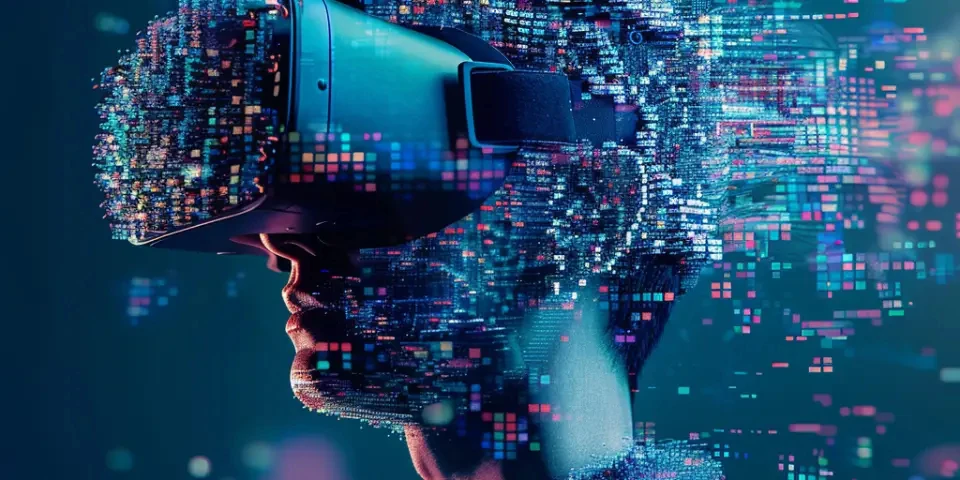
1. Data-driven Decision Making
AI algorithms have the ability to analyze vast amounts of data from diverse sources, allowing businesses to make data-driven decisions. By using AI-powered tools and platforms, businesses can gather and process data from social media, customer surveys, industry reports, and other relevant sources to identify patterns and trends in consumer behavior. This empowers businesses to anticipate market shifts and make proactive decisions based on accurate insights.
For example, a retail company could use AI algorithms to analyze customer purchase patterns and preferences to predict future trends in consumer demand. This can help them optimize their inventory and marketing strategies, ensuring they have the right products at the right time to meet customer expectations.
2. Sentiment Analysis
Sentiment analysis is a powerful AI application that can gauge public opinion about products, brands, or market conditions. By analyzing social media posts, online forums, and customer reviews, businesses can gain valuable insights into consumer sentiment and identify emerging trends. Sentiment analysis can be particularly useful for assessing the success of marketing campaigns, identifying potential issues with products or services, and predicting consumer behavior.
For instance, a hotel chain could use sentiment analysis to monitor customer reviews and identify trends in customer satisfaction. By analyzing sentiment data, they can make necessary improvements to enhance the customer experience and stay ahead of competitors.
3. Machine Learning Algorithms
Machine learning algorithms are at the core of AI-driven predictive analytics. These algorithms can analyze historical data, identify patterns, and make predictions about future events or trends. Machine learning models can adapt and improve over time as they are exposed to more data, enabling businesses to make increasingly accurate forecasts.
For example, a financial institution could use machine learning algorithms to analyze historical market data and predict future stock market trends. By understanding market fluctuations and identifying potential investment opportunities, the institution can guide their investment strategies and gain a competitive advantage in the market.
4. Natural Language Processing
Natural Language Processing (NLP) is a branch of AI that focuses on the interaction between computers and humans through natural language. NLP-powered tools can analyze text data from various sources, including news articles, research papers, and customer feedback, to extract meaningful insights.
An example of utilizing NLP for predicting market trends is a news analytics platform that uses NLP algorithms to analyze news articles related to specific industries. By extracting key information from these articles, the platform can provide businesses with real-time insights, helping them anticipate market shifts and make informed decisions.
5. Competitive Intelligence
AI can also be used to gather and analyze competitive intelligence, providing businesses with invaluable insights into their competitors' strategies, products, and market positioning. By examining publicly available data, including websites, social media, and press releases, AI-powered tools can identify competitors' strengths and weaknesses, allowing businesses to strategically position themselves in the market.
An AI-driven competitive intelligence platform could, for example, aggregate and analyze pricing information from various e-commerce websites to help businesses set competitive prices and optimize their revenue.
6. Forecasting Demand
Accurate demand forecasting is essential for businesses to optimize production, inventory, and supply chain management. AI can analyze historical sales data, economic indicators, and other relevant factors to predict future demand and facilitate efficient resource allocation.
A manufacturing company could use AI-powered demand forecasting models to predict spikes or declines in demand for their products. This allows them to adjust production levels and ensure optimal inventory management, avoiding shortages or excess stock.
7. Risk Assessment
A predictive AI model can assess potential risks and their impact on business operations. By analyzing historical data and external factors like economic indicators, market volatility, and geopolitical events, businesses can proactively identify and mitigate risks.
For instance, an insurance company could use AI algorithms to analyze customer data and identify patterns of potential fraudulent behavior. This helps them minimize risk and protect their business from financial losses.
8. Customer Segmentation
AI-driven customer segmentation enables businesses to categorize their customer base into distinct groups based on their behaviors, preferences, and purchasing patterns. By understanding how various customer segments are likely to respond to different market trends, businesses can tailor their marketing strategies and offerings to maximize customer engagement and loyalty.
A retail company could use AI algorithms to segment their customer base and personalize their marketing campaigns accordingly. By aligning their offerings with customer preferences, they can create personalized experiences that drive customer loyalty and gain a competitive edge.
Conclusion
As AI continues to advance, its potential to predict future market trends grows exponentially. By leveraging the power of data and AI-driven predictive analytics, businesses can make informed decisions, stay ahead of the competition, and capitalize on emerging opportunities. However, it is important to recognize that while AI can provide valuable insights, human intelligence and expertise are still crucial in interpreting and applying these insights effectively.
Frequently Asked Questions
Q1: Can any business benefit from AI-driven predictive analytics?
A1: Yes, businesses across various industries can benefit from AI-driven predictive analytics. However, the extent of the benefits may vary depending on the availability and quality of data, the complexity of the industry, and the specific business goals.
Q2: Are there any ethical concerns associated with AI predictions?
A2: Yes, ethical concerns such as data privacy, bias in algorithmic decision-making, and potential job displacement need to be addressed when implementing AI-driven predictive analytics. It is crucial for businesses to ensure they have robust data governance policies and practices in place.
Q3: What are the challenges of implementing AI-driven predictive analytics?
A3: Some of the challenges include data quality and availability, integration with existing systems and processes, technical expertise in AI implementation, and understanding the limitations and potential biases of AI models.
References
1. Smith, J. (2019). Artificial intelligence in marketing: What you need to know. HubSpot. Retrieved from [link]
2. Bharti, S.K. et al. (2020). Predictive analytics in business: Issues and challenges. International Journal of Advanced Science and Technology, 29(06), 3047-3057.
3. Petropoulos, G. et al. (2017). Forecasting market-level dynamics using real-time internet search information. Journal of Business Research, 79, 239-246.
Explore your companion in WeMate