Transforming Synthetic Biology Research with AI-driven Experimental Planning

Synthetic biology, an interdisciplinary field that combines biology and engineering, has the potential to revolutionize industries ranging from healthcare to agriculture. However, the complexity of biological systems often poses challenges in experimental design and data analysis. As a solution, artificial intelligence (AI) is emerging as a powerful tool to transform synthetic biology research by guiding and optimizing experimental planning.
1. AI-powered Design of Genetic Constructs
One of the key challenges in synthetic biology is designing optimal genetic constructs for desired functions. AI algorithms can analyze vast biological databases to predict the behavior of different genetic elements, helping researchers design constructs with desirable traits. Tools like the Genetic Constructor by Twist Bioscience and Benchling's DNA design platform leverage AI-driven algorithms to streamline and optimize the process.
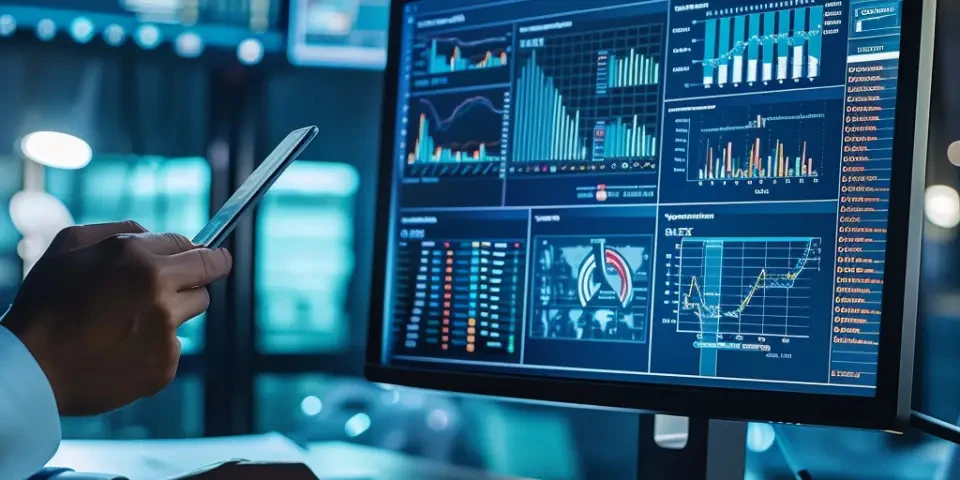
AI can also assist in identifying potential design flaws by analyzing the stability, toxicity, and expression levels of different genetic elements. This enables researchers to fine-tune their constructs and enhance their functionality before experimental implementation.
2. Enhanced Prediction of Biological Interactions
Understanding the interactions between genetic elements, proteins, and cellular pathways is crucial in synthetic biology research. AI algorithms can integrate diverse genomic and proteomic datasets to predict these interactions more accurately, reducing the need for time-consuming laboratory experiments.
Through machine learning algorithms, AI can identify patterns in biological data and generate models that predict how different components will interact within a living system. This enables researchers to make informed decisions about which experiments to prioritize and rapidly iterate their designs.
3. AI-guided High-throughput Experimentation
Synthetic biology experiments often involve testing thousands of genetic variations or conditions, making it impractical for researchers to manually plan and execute each experiment without errors. AI-driven experimental planning platforms, such as Transcriptic and Synthace's Antha, enable high-throughput experimentation by automating experimental design and execution.
These platforms utilize AI algorithms to optimize experimental parameters, such as sample size, incubation time, and reagent volumes, maximizing data generation while minimizing resource utilization. By efficiently exploring large experimental spaces, researchers can uncover valuable insights more rapidly and accelerate the discovery of novel biological designs.
4. Data Integration and Analysis
With the advancement of AI, handling and analyzing large-scale biological datasets has become more manageable. AI algorithms can integrate data from various sources, such as omics datasets, literature repositories, and experimental results, to provide a comprehensive understanding of biological systems.
Through AI-powered data integration and analysis platforms like Network Medicine Platform (NeMo) and GeneMANIA, researchers can identify key genes, protein-protein interactions, and pathways involved in specific biological processes. These insights can guide experimental design and aid in uncovering hidden relationships and potential targets for further investigation.
5. Optimization of Biological Processes
Optimizing biological processes, such as metabolic pathways or protein production, is essential in synthetic biology. AI-driven optimization algorithms, such as genetic algorithms and reinforcement learning, can analyze the vast parameter space of biological systems to identify optimal conditions for desired outputs.
By leveraging these algorithms, researchers can maximize desired product yields while minimizing unwanted byproducts or energy consumption. This can have significant implications for industries like biofuels, pharmaceuticals, and agriculture, where the efficient production of valuable compounds is crucial.
6. AI-guided Protein Design
Protein engineering plays a vital role in synthetic biology, allowing researchers to design enzymes with specific functions or properties. AI algorithms, such as deep learning and generative models, can predict protein structures, functions, and interactions based on available data.
Tools like Rosetta and Alphafold utilize these algorithms to assist in protein design by generating potential structures and optimizing properties, such as stability or binding affinity. This AI-guided approach accelerates the design process, enabling the creation of novel proteins for various applications, including drug development and industrial biotechnology.
7. AI-assisted Biomaterial Design
Synthetic biology extends beyond genetic engineering to the design of biomaterials with tailored properties. AI-driven materials design tools, such as the Materials Project and Citrine Informatics, leverage machine learning algorithms to predict and optimize material properties based on composition, structure, and processing conditions.
These AI-guided platforms enable researchers to discover new biomaterial compositions and optimize their characteristics, such as mechanical strength, biocompatibility, or degradation rates. Through the rational design of biomaterials, synthetic biology is poised to deliver breakthroughs in tissue engineering, regenerative medicine, and drug delivery systems.
8. AI-driven Biosafety and Biosecurity
While synthetic biology holds tremendous promise, ensuring biosafety and biosecurity is of paramount importance. AI can play a vital role in predicting the potential risks associated with genetic constructs and engineered organisms.
By analyzing genomic sequences and comparing them against known databases, AI algorithms can identify sequences with potential biosafety concerns, such as toxin production or pathogenicity. Tools like GeneChecker and DNAlytics aid in screening genetic constructs and evaluating potential risks, facilitating responsible research and development practices in synthetic biology.
Frequently Asked Questions (FAQs):
1. Can AI replace human researchers in synthetic biology?
No, AI cannot replace human researchers in synthetic biology. AI is a powerful tool that aids researchers in experimental planning, data analysis, and design optimization. Human expertise is still required for interpreting results, making critical decisions, and generating novel hypotheses.
2. Are there any ethical concerns associated with AI-driven synthetic biology research?
Yes, the convergence of AI and synthetic biology raises ethical concerns surrounding safety, security, and responsible use. These concerns must be addressed through robust regulation, transparent communication, and a multidisciplinary approach involving experts from various fields.
3. How accessible are AI-driven tools in synthetic biology research?
While some AI-driven tools are commercially available, the accessibility varies. The field is rapidly evolving, and more user-friendly platforms are being developed. Collaborations between academic institutions, industry, and open-source communities are crucial in democratizing AI-driven tools and making them widely accessible.
References:
1. Johnson, G. R., & Beil, S. (2020). Applications of artificial intelligence in synthetic biology. Current Opinion in Systems Biology, 19, 12-19.
2. Carbonell-Ballestero, M., Garcia-Ramallo, E., Montanez, R., & Rodriguez-Caso, C. (2021). Artificial intelligence in synthetic biology: a review. Synthetic Biology, 6(1), ysaa019.
3. Cheah, A. M., Brito, I. L., & Blum, M. (2021). AI-guided design of synthetic microbial communities. Trends in Biotechnology, 39(8), 812-826.
Explore your companion in WeMate