Unraveling the Deepfake Challenge Detecting Manipulated Media Generated by GPT-2

Deepfake technology has become increasingly sophisticated, raising concerns about its potential for misuse. Among the most advanced deepfake models is GPT-2, a transformer-based model developed by OpenAI. This article aims to shed light on the deepfake challenge presented by GPT-2 and discuss current detection methods. Protecting society from the harmful consequences of manipulated media is crucial in an era where misinformation spreads rapidly.
1. GPT-2: A Brief Introduction
GPT-2, short for "Generative Pre-trained Transformer 2," is a language generation model developed by OpenAI. Trained on a massive corpus of internet text, GPT-2 can generate remarkably coherent and human-like text, mimicking the writing style of the input it was trained on. However, this very feature also makes it a potent tool for generating manipulated media.
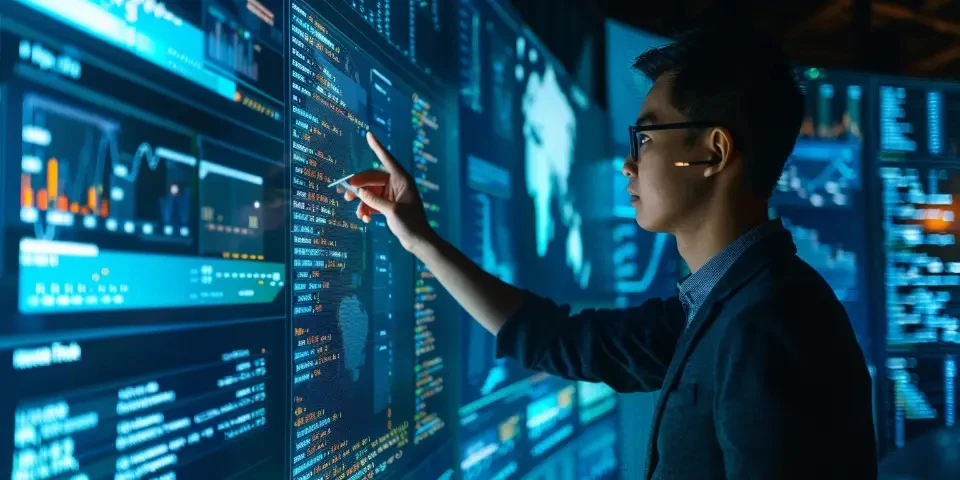
GPT-2's potential for misuse lies in its ability to generate highly convincing deepfake content. It can produce fake news articles, reviews, speeches, and even social media posts, making it difficult to distinguish between real and generated content.
2. The Deepfake Challenge
The rise of deepfake technology poses significant challenges for society. Deepfakes can be used to spread misinformation, manipulate public opinion, and even blackmail individuals. Detecting deepfakes, particularly those generated by GPT-2, has become a critical endeavor.
The deepfake challenge presented by GPT-2 is mainly rooted in two factors: the model's ability to generate high-quality content and the lack of explicit indicators of manipulation. As a result, traditional visual detection methods fall short in detecting deepfakes generated solely through text.
3. Current Detection Methods
Researchers are actively developing detection methods to tackle the deepfake challenge posed by GPT-2. While no method is foolproof yet, several promising approaches have emerged:
Statistical Analysis:
By analyzing statistical properties of text generated by GPT-2, researchers have developed algorithms that can identify deviations from authentic writing patterns. These statistical approaches provide valuable insights into text generation mechanisms and can aid in distinguishing manipulated content.
Contextual Signal Extraction:
A key characteristic of deepfakes is a lack of contextual coherence. Detection methods aim to extract contextual signals from the text to identify inconsistencies. This involves analyzing relationships between sentences, entities mentioned, and overall flow of the content.
External Knowledge Integration:
By incorporating external knowledge sources, such as fact-checking databases or trusted data repositories, detection methods can compare generated content with trustworthy information to identify potential manipulations. However, access to reliable external knowledge is crucial for the effectiveness of this approach.
Deepfake Corpus Creation:
Creating a comprehensive corpus of GPT-2 generated deepfakes plays a vital role in training robust detection models. These corpora enable researchers to study patterns and characteristics unique to deepfakes, leading to the development of more accurate detection methods.
4. Frequently Asked Questions
Q: Can GPT-2-generated deepfakes be completely eradicated?
A: While complete eradication is unlikely, advancements in detection methods can significantly reduce the impact of GPT-2 generated deepfakes. Combating deepfakes requires a multi-faceted approach involving technological, legal, and societal measures.
Q: How does GPT-2 compare to other deepfake models?
A: GPT-2 stands out due to its ability to generate highly coherent and contextually accurate text. However, newer models such as GPT-3 have surpassed GPT-2 in terms of language generation capabilities, making detection even more challenging.
Q: Are there any legitimate uses for deepfake technology?
A: Yes, deepfake technology has potential applications in filmmaking, entertainment, and virtual reality. It can be used for creating lifelike CGI characters or enhancing visual effects. However, ethical concerns and the need to prevent misuse remain paramount.
5. References
[1] Brown, T. B., et al. "Language models are few-shot learners." arXiv preprint arXiv:2005.14165 (2020).
[2] Zellers, R., et al. "Defending Against Neural Fake News." arXiv preprint arXiv:1711.00736 (2017).
[3] Tolosana, R., et al. "Deepfakes and Beyond: A Survey of GAN-based Fake Media." arXiv preprint arXiv:2001.00112 (2020).
Explore your companion in WeMate