The Ethical Dilemma Addressing Bias and Transparency in AI Systems

Artificial Intelligence (AI) systems have been transforming various industries, revolutionizing how we live, work, and interact. However, the rapid advancement and implementation of AI technology also raise important ethical dilemmas. Two significant concerns that need to be addressed are bias and transparency within AI systems. This article delves into the implications of bias and lack of transparency, explores the challenges involved, and proposes potential solutions to ensure the ethical use of AI.
Bias in AI Systems
1. Bias in Training Data:
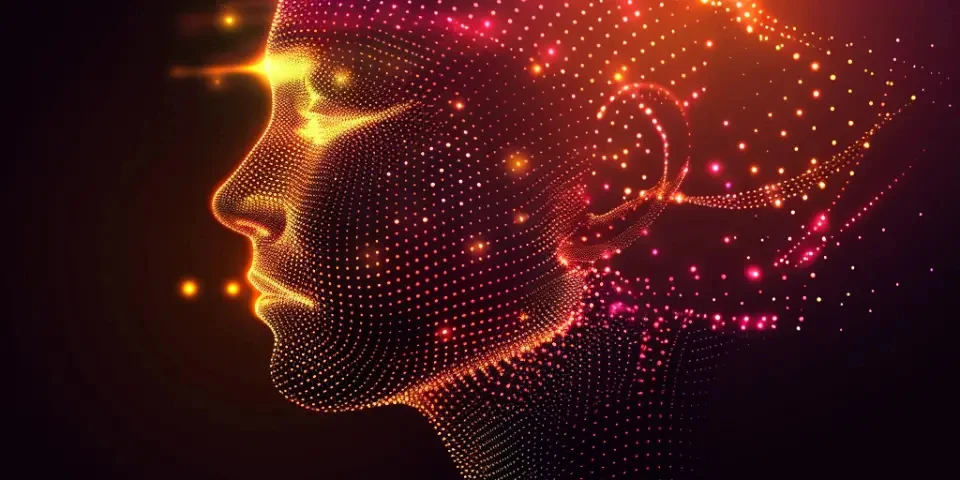
AI systems rely heavily on training data to make informed decisions. However, if the training data is biased, the system may unintentionally perpetuate and amplify existing societal biases. For instance, if a facial recognition AI system is trained predominantly on data from light-skinned individuals, it might struggle to accurately recognize individuals with darker skin tones.
2. Consequences of Biased Decisions:
When AI systems incorporate biased training data, they generate biased outcomes that can have profound consequences. Biased decisions in hiring processes or loan approvals, for example, can perpetuate discrimination against specific genders, races, or socioeconomic groups, thus reinforcing societal inequalities.
Transparency in AI Systems
1. Black Box Problem:
AI systems often function as "black boxes," making decisions without providing clear explanations for their outcomes. This lack of transparency raises concerns about accountability, as it becomes challenging to understand and challenge the reasoning behind certain decisions made by AI systems.
2. Understanding and Trust:
Transparency is crucial to building trust in AI systems. Without clear explanations of how AI systems reach their conclusions, users may be hesitant to rely on them for critical decisions, leading to limited acceptance and adoption.
Challenges in Resolving Bias and Transparency Issues
1. Data Collection and Representation:
Collecting unbiased and representative data is a formidable challenge, as historical data often reflects societal biases. Developing techniques to identify and correct these biases without introducing new ones is a demanding task.
2. Trade-Off between Accuracy and Ethics:
Striking a balance between accurate AI predictions and eliminating bias is complex. Adjusting AI systems to avoid biased outcomes may reduce accuracy, underscoring the need for careful calibration.
Potential Solutions
1. Diverse and Inclusive Data:
Using diverse and inclusive datasets during AI training can help reduce bias in system outcomes. By incorporating perspectives from various demographics, the AI system becomes more robust and avoids discrimination against underrepresented groups.
2. Regular Auditing and Testing:
Establishing regular audits and testing frameworks can ensure transparency and identify potential biases within AI systems. These audits should involve external experts to provide an impartial assessment of the system's fairness and accuracy.
3. Explainable AI (XAI):
Developing AI systems that provide understandable explanations for their decisions would improve transparency and increase user trust. XAI techniques, such as generating visual or language-based explanations, can aid in understanding the inner workings of AI systems.
Frequently Asked Questions
Q1: Can bias in AI systems be completely eliminated?
A1: While it may be challenging to eliminate bias entirely, significant efforts can be made to minimize its impact. Continuous monitoring, auditing, and diversifying training data are key steps towards reducing bias in AI systems.
Q2: How can AI bias be detected?
A2: AI bias can be detected through thorough testing and evaluation of system outputs. By comparing outcomes across various demographic groups, any discriminatory patterns can be identified and addressed.
References
1. Smith, B. R., & Anderson, M. (2020). Artificial intelligence bias: how does it happen and how can we address it? BMC Medical Informatics and Decision Making, 20(1), 1-10.
2. Mittelstadt, B. D., Allo, P., Taddeo, M., Wachter, S., & Floridi, L. (2016). The ethics of algorithms: Mapping the debate. Big Data & Society, 3(2), 2053951716679679.
Explore your companion in WeMate